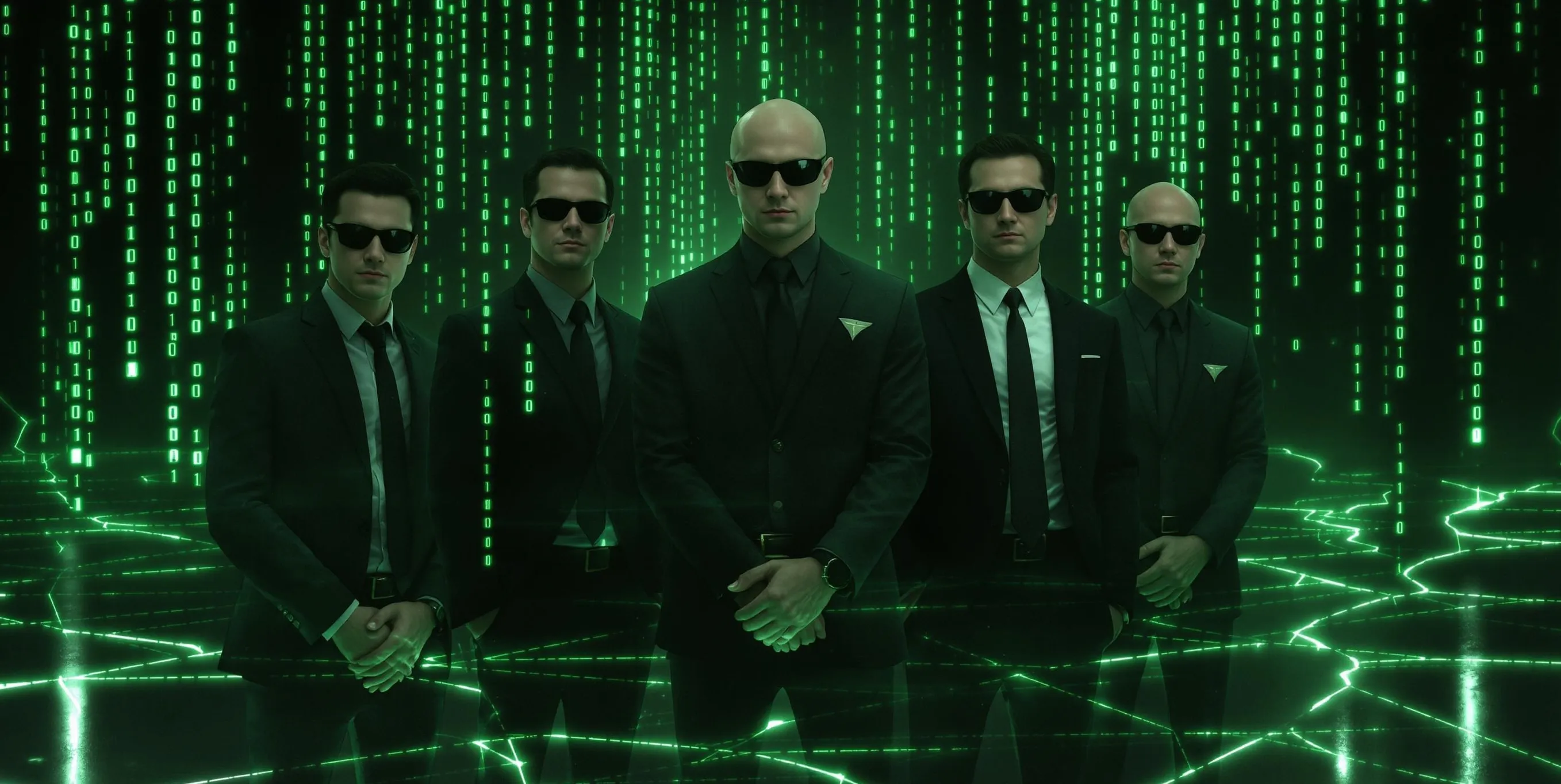
What on Earth are Agents?
/ 21 min read
The year 2024 marked a turning point for AI, dominated by Retrieval-Augmented Generation (RAG). With groundbreaking models like GPT-4o 1, Claude-3.5-Sonnet 2, and Llama 3.1 3 widely available for adoption, the industry raced to harness ever-larger language models for enterprise use cases. Yet a critical question lingered:
How do we transform these models into adaptable tools that deliver real-world business value?
At SAP, teams within our organisation explored this challenge firsthand, building sophisticated RAG architectures to streamline workflows and empower employees and customers through Generative AI application grounded with proprietary data. While these systems proved transformative for various business operations, they still operated within rigid, predefined boundaries – a limitation that sparked a broader industry reflection.
By late 2024, a new paradigm emerged: agentic AI. Everyone’s favourite AI mentor – Andrew Ng placed a big bet on agentic AI 4 and the same was validated as an emerging topic in Deloitte’s 2024 State of Generative AI Report 5. This approach reimagined AI systems as autonomous agents capable of reasoning, planning, and acting with human-like adaptability. No longer confined to retrieving and regurgitating information, these agents promised to make decisions, collaborate in multi-agent ecosystems, and dynamically respond to unscripted scenarios – a leap forward that resonated deeply with enterprise needs.
In this article, we’ll dissect the architecture of modern AI agents, exploring:
- Core principles separating agents from traditional RAG systems
- Emerging design patterns reshaping enterprise AI strategies
- Real-world applications of agents across industries
- Critical considerations for building robust, ethical agentic workflows
What is an LLM Agent? An AI Industry Perspective
While definitions vary, AI agents share a core trait: autonomy. Unlike traditional AI systems that follow rigid workflows, agents dynamically perceive, reason, plan, and act to achieve goals with minimal human intervention. Let’s dissect this through the lens of key players in foundation models and LLM orchestration:
- OpenAI: Agentic AI systems as the ability to take actions towards a specified goal autonomously without having to be explicitly programmed 6. They liken agents to be like Samantha from Her 7 and HAL 9000 from 2001: A Space Odyssey 8.
Agenticness – their coined metric for an AI’s ability to self-direct without explicit instructions.
- Google: Emphasizes tool-wielding problem-solvers – agents observe environments (data streams, APIs) and act using available resources 8.
- Anthropic: Agents can be viewed as agentic systems that are either architected as workflows, where LLMs and tools are integrated through predefined logic, or as dynamic systems in which LLMs make their own decisions with a certain degree of autonomy. 9.
- LangChain: Views agents in a more technical light, as a system that uses LLM to decide how an application should flow 10.
LangChain’s perpective argues that agents capabilities can be view as a spectrum from being a simple State Machine to a full-fledged Autonomous system.
- LlamaIndex: Agents as a “automated reasoning and decision engine” that make internal decisions to solve tasks 11.
- CrewAI: Agents as piece of software autonomously take decisions towards accomplishing a goal 12.
The Big Picture: Definitions stretch from sci-fi aspirations (OpenAI’s Samantha) to pragmatic tooling (LangChain). What unites them? A shared focus on shifting AI from rigid “do what I say” executors to adaptive “figure it out” collaborators.
Key Takeaway: Agents = LLMs + Tools + Decision-Making Loops.
Although we’ve observed a paradigm shift from RAG to AI agents, it’s important to recognize that these applications of LLMs are not mutually exclusive. In fact, Agentic RAG 13 serves as a prime example of integrating agents within the RAG framework.
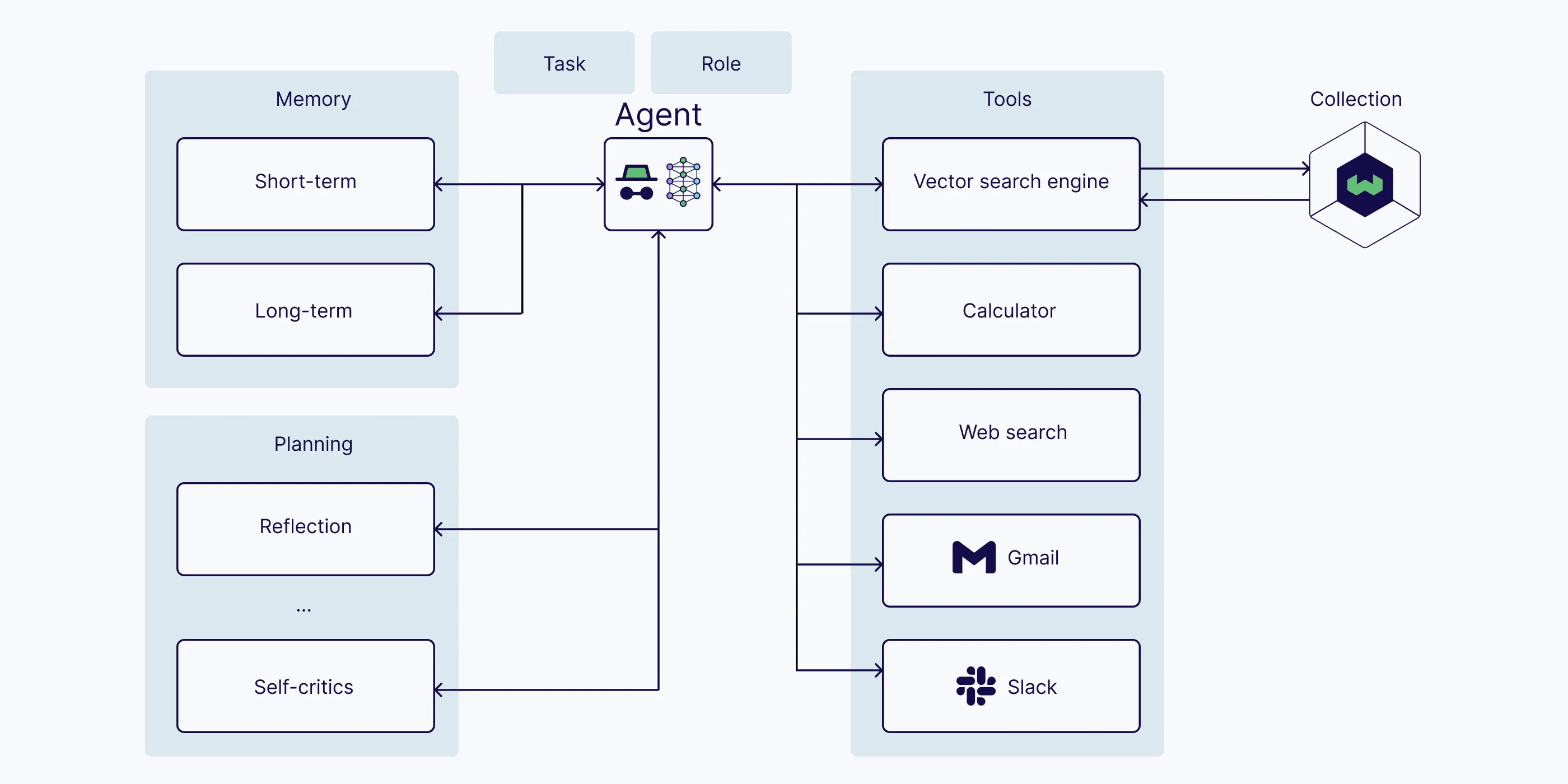
Unlike traditional RAG, which follows a one-shot retrieval and response process, Agentic RAG introduces reasoning and autonomous decision-making, enabling more adaptive and iterative retrieval strategies for improved outcomes.
Common Agentic Design Patterns
When developing software, it is essential to understand widely adopted design patterns that enhance scalability, maintainability, and efficiency. Similarly, in the field of AI, building new models requires a solid grasp of common architectural designs, core components, and optimization techniques.
The same principles apply to agent-based systems. To create effective and interpretable agentic AI workflows, it is crucial to explore various agent design patterns that can be tailored to different scenarios, ensuring adaptability and optimal performance.
These patterns act as building blocks, enabling systems that are:
Principle | How Patterns Enable It | Example |
---|---|---|
Modularity | Patterns represent reusable modules (e.g., a “reflection” module for self-correction). | Swap a symptom-classifier agent in a medical system without disrupting the diagnosis workflow. |
Scalability | Patterns compose hierarchically (e.g., planning agents orchestrating sub-agents). | Scale a customer service system from handling 10 to 10,000 queries by adding parallel agents. |
Interpretability | Clear patterns map to human-understandable processes (e.g., financial agent works on financial processes). | Audit why a financial agent rejected a loan by tracing its decision process. |
Flexibility | Mix and match models/tools (e.g., GPT-4 for creativity, Claude for safety checks). | Deploy a coding agent with Claude-3.5-Sonnet for syntax and OpenAI’s o1 for high-level design. |
The following design patterns discussed are not meant to be exhaustive but rather serve as a starting point for understanding the diverse landscape of agentic AI systems.
Reflection
When we think of reflection, we often associate it with introspection or self-awareness. Similarly, in AI agents, reflection refers to the ability to analyze actions, decisions, and outcomes to improve future performance.
Reflection: Enhances an agent’s reasoning through iterative self-analysis and self-correction.
This process is critical in scenarios requiring alignment with rules or learning from mistakes. For example, a content generation agent might draft text, analyze it against marketing guidelines (e.g., compliance with regulations), detect mismatches, and iteratively revise until alignment is achieved. Unlike static systems, reflection agents adapt dynamically, turning past errors into opportunities for refinement.
Basic Reflection
The basic design pattern for reflection involves a feedback loop: the agent analyzes its actions and outcomes to refine future decisions.
The reflection process can range from simple to complex. For example:
- Simple: A customer service agent compares generated text against a set of rules (e.g., avoiding offensive language) encoded in the original query and revises its response.
- Complex: A financial trading agent analyzes market trends, historical trades, and news sentiment to identify patterns and adjust its strategy.
While implementations vary, the core idea is to iterate until the desired outcome is achieved. Each cycle allows the agent to incorporate new insights, reducing errors and improving alignment with goals.
We’ll explore advanced implementations of this concept (like Reflexion, which integrates tool usage) in the next pattern.
Reflexion
Reflexion enhances basic reflection by integrating external tools into the feedback loop. These tools act as objective validators, providing additional context (e.g., data, rules, domain-specific knowledge) to critique and verify the agent’s decisions. Think of it as consulting a trusted advisor or reference material for a second opinion.
Core Idea: Reflexion agents explicitly critique their own decisions and ground responses in external validations or data, ensuring objectivity and reducing bias.
As I may be providing an oversimplified explanation of the pattern, please refer to the original paper for deeper insights 14.
Example Workflow:
- A medical diagnosis agent generates a list of potential conditions based on symptoms.
- It consults a clinical database to cross-validate recommended treatments against the patient’s medical history.
- If conflicts arise (e.g., a drug allergy), the agent revises its recommendations iteratively until alignment is achieved.
Basic reflection and reflexion patterns form the foundation for agents to self-correct and improve over time. However, these are just the beginning. Other advanced design paradigms – rooted in the same principles of iterative analysis – address even more complex challenges, such as multi-step reasoning, collaborative problem-solving, or optimizing decision trees. For example:
- Language Agent Tree Search (LATS) 15: Combines reflection with tree-search algorithms (like those used in game-playing AI) to simulate and evaluate multiple reasoning paths before committing to a final decision.
- Multi-Agent Debate: Enables multiple AI agents to critique each other’s outputs iteratively, refining solutions through structured “discussion.”
To dive deeper into these patterns, I recommend watching this insightful video on “Reflection Agents” by LangChain:
Tool Use
While modern LLMs demonstrate impressive (arguable) reasoning capabilities, their true power in real-world applications often lies in integrating external tools to overcome inherent limitations.
Why Tools Matter:
- Access to Proprietary/Private Data: LLMs lack knowledge of internal company data (e.g., CRM records, proprietary research). Tools like database connectors or APIs let agents retrieve this information dynamically.
- Real-Time Updates: LLMs are frozen in time post-training. Tools like web search APIs or live data feeds enable agents to incorporate current events, stock prices, or weather forecasts.
- Domain-Specific Validation: Tools like code compilers, fact-checking databases, or compliance validators ensure outputs meet technical or regulatory standards.
Example Workflows:
- Customer Service Agent:
- Problem: An LLM might misstate a user’s order history if trained on generic data.
- Solution: Integrate a tool to query the company’s internal database, enabling personalized, accurate responses.
- Financial Analyst Agent:
- Problem: An LLM can’t natively track real-time market shifts.
- Solution: Use a stock market API to fetch live data, then analyze trends to recommend portfolio adjustments.
Tool use transforms LLMs from static knowledge repositories into dynamic problem-solvers capable of context-aware, up-to-date reasoning.
While tool integration isn’t new (e.g., RAG uses databases to fetch context for responses), agents take tool usage a step further.
RAG | Agents |
---|---|
Tools retrieve information to inform a response. | Tools drive decision-making during reasoning (e.g., validating hypotheses, running calculations, triggering actions). |
Static: Tools are used once to gather context. | Dynamic: Tools are invoked iteratively as part of the reasoning process. |
Example: Querying a knowledge base to answer “What’s the capital of France?” | Example: Using a calculator to optimize a budget, calling an API to book a flight, or validating code syntax before execution. |
Planning
When we think of planning, we often associate it with project management or strategic decision-making. In AI agents, planning refers to the ability to decompose complex tasks into actionable steps, sequence them logically, and adaptively execute them – even in uncertain environments.
Planning: The systematic process of breaking down objectives into smaller tasks, orchestrating their execution, and dynamically adjusting based on feedback.
Planning is crucial for agents tackling multi-step problems where a single response is insufficient. It helps agents avoid infinite loops that could prevent them from achieving the desired outcome. Additionally, planning ensures that agents consider all critical steps – for example, assisting in medical diagnosis while also verifying drug interactions when recommending treatment plans.
Plan-and-Execute
Inspired by BabyAGI, the plan-and-execute pattern emphasizes multi-step planning followed by sequential execution, with re-planning triggered by feedback from completed tasks.
First, the system generates an initial plan (e.g., breaking a goal into subtasks like “Research → Analyze → Summarize”). It then executes these tasks one by one, dynamically adjusting the plan if intermediate results deviate from expectations – such as incomplete data, errors, or new constraints.
This mirrors the process of following a recipe: a chef first outlines steps (chopping, sautéing, baking) but adapts if ingredients burn or substitutions are needed, ensuring the final dish still meets the desired outcome.
Further examples can be summarized as follows:
Scenario | Plan-and-Execute Workflow |
---|---|
Content Creation | Plan: Outline blog sections → Execute: Write each section → Re-plan: If topic shifts. |
Project Management | Plan: Define milestones → Execute: Assign tasks → Re-plan: If deadlines slip. |
Software Development | Plan: Architecture design → Execute: Code modules → Re-plan: If bugs block progress. |
LLMCompiler
Building on the plan-and-execute pattern, LLMCompiler introduces parallel task execution through a dynamically generated Directed Acyclic Graph (DAG). Instead of processing tasks sequentially, the pattern identifies independent tasks and eagerly streams them for concurrent execution, significantly accelerating workflows.
How It Works:
- DAG Construction: Breaks down objectives into tasks with explicit dependencies (e.g., “Task B depends on the output from Task A”).
- Parallel Execution: Executes tasks as soon as their dependencies are resolved (e.g., Tasks C and D can run simultaneously if neither depends on the other).
- Joiner Component: Aggregates and validates outputs from parallel tasks, flagging inconsistencies (e.g., conflicting data from two research agents).
- Re-planning Loop: If errors or mismatches occur, the system re-enters planning mode to adjust the DAG (e.g., adding a new “data reconciliation” task).
While plan-and-execute follows a sequential, step-by-step process (like a single chef preparing a dish), LLMCompiler is more like a kitchen crew working in parallel to prepare the same dish simultaneously, accelerating the process and improving efficiency.
Multi-Agent
In the real world, solving complex problems – like launching a rocket or developing a vaccine – requires experts with diverse skills to collaborate. Similarly, multi-agent collaboration in AI orchestrate specialized agents, each with distinct roles and expertise, to tackle challenges collectively.
But why use multiple agents instead of a single agent?
Firstly, scalability is a key factor. With multiple agents, we can divide complex tasks into smaller, manageable sub-tasks, enabling parallel processing and faster problem-solving. Secondly, specialization is another advantage. Each agent can focus on a specific aspect of the problem, leveraging its unique capabilities to contribute to the overall solution. Thirdly, robustness is enhanced. If one agent fails or makes an error, other agents can compensate, ensuring the system continues to function effectively.
Multi-Agent Systems: Collaborative networks of agents pooling resources, tools, and expertise to solve complex problems through division of labor and shared reasoning.
Example: Medical Diagnosis Team
- Symptom Classifier: Identifies patterns in patient-reported symptoms.
- Drug Interaction Checker: Cross-references medications for contraindications.
- Treatment Recommender: Proposes therapies aligned with clinical guidelines.
- Coordinator Agent: Synthesizes inputs, resolves conflicts (e.g., via debate), and finalizes the care plan.
This collaboration mimics a hospital team rounding on a patient – each specialist contributes insights, while a lead physician integrates findings. Multi-agents collaboration synergises with previously discussed agentic patterns:
- Reflection: Agents critique each other’s outputs, refining solutions.
- Tool Use: Agents leverage shared tools (e.g., medical databases, drug interaction checkers).
- Planning: Tasks are divided and sequenced (e.g., “First diagnose, then check interactions, then recommend treatments”).
In the overall context of AI agents, multi-agent system is a powerful design pattern that enables agents to tackle complex problems beyond the scope of individual LLMs or agent.
Collaboration
Building on the collaboration pattern, we can explore more specialized multi-agent architectures that require a nuanced division of labor. The collaboration pattern serves as a fundamental framework for multi-agent systems, offering a structured way to distribute tasks among agents.
For example, when generating a new script for e.g., data processing, the collaboration pattern can break down the process into specific sub-tasks, such as code writing and bug fixing:
- Code-writer Agent: Analyse requirements and generate code snippets/script.
- Bug-fixer Agent: Validate code, identify and fix errors.
Each agent specializes in a distinct task, working in tandem to produce the final code snippet/script efficiently.
While the collaboration pattern provides structure and coordination, it can often be perceived as more “rigid” compared to the autonomous multi-agent pattern, which offers greater flexibility and independence among agents.
Supervisor
The supervisor pattern introduces a hierarchical structure to multi-agent collaboration, where a lead agent (supervisor) oversees and coordinates the activities of sub-agents. This pattern is particularly effective in scenarios that require centralized task management, ensuring efficient delegation and coordination among specialized agents.
By transitioning from a flat collaborative model to a hierarchical structure, the supervisor pattern enhances the organization and management of multi-agent systems. It empowers the AI system with greater autonomy to oversee its internal processes, optimizing resource allocation and workflow efficiency.
Aspect | Collaboration Pattern | Supervisor Pattern |
---|---|---|
Structure | Flat, peer-to-peer interaction | Hierarchical (supervisor → sub-agents) |
Task Delegation | Sequentially, often based on task workflow | Supervisor assigns (route) tasks based on sub-agent expertise, autonomy on agents |
Autonomy | Tasks are shared sequentially based on workflow | Sub-agents focus on execution; supervisor manages workflow |
Hierarchical
While multi-agent collaboration offers a significant shift from single-agent systems, enabling us to tackle complex problems, challenges can still arise when individual agents struggle with sub-problems that may remain too intricate to resolve independently.
In such cases, a deeper hierarchical structure can be introduced within the supervisor pattern. Here, a lead agent (supervisor) oversees and coordinates sub-supervisor agents, who, in turn, manage their respective sub-agents.
Sounds complex? Let’s relate this to a real-world scenario:
Imagine an organization working on a major proposal. The organization may be divided into pillars, with each pillar further broken down into teams, each led by a team lead and consisting of members with diverse expertise.
For instance:
- The research team might divide their work into multiple sub-topics, each requiring specialized knowledge and further delegation.
- Once the research is complete, the proposal writing team may split the document into various sections, with different members focusing on specific parts.
- Similarly, other teams involved in the project will follow their own structured breakdown to ensure a comprehensive and efficient approach.
Hierarchical pattern enhances coordination and ensures that complex tasks are handled effectively, leveraging specialized expertise at multiple levels.
Human-in-the-Loop
In an ideal agentic AI system, we aim to grant full autonomy to agents to handle tasks of varying complexity. However, through experimentation, we often discover occasional inaccuracies that can impact performance.
In business-critical operations – such as compliance and key decision-making processes – where AI may be introduced to enhance capabilities, it becomes crucial to maintain accuracy and reliability. To address this, the human-in-the-loop pattern can be implemented. This approach allows human intervention to review, correct, and override certain agent decisions when necessary.
In an agentic AI system, human intervention can occur in the following areas:
- Tool Calls: Validate, edit, and approve the usage of tools by agents.
- Agent Outputs: Validate, edit, and approve outputs generated by agents at various stages of the process.
- Context: Provide additional information to agents, enhancing their ability to support subsequent stages of decision-making or task completion.
By incorporating human oversight, organizations can strike a balance between AI-driven efficiency and the assurance of human judgment, ensuring that critical processes remain accurate, transparent, and aligned with business objectives.
An example of when to use the human-in-the-loop pattern can be observed as follows:
Scenario | HITL Recommended? | Rationale |
---|---|---|
Legal contract drafting | ✅ | Avoid liability risks from AI missing nuanced clauses |
Inventory management | ❌ | Fully autonomous agents optimize restocking efficiently |
Patient diagnosis support | ✅ | Ensure alignment with evolving medical best practices |
Real-World Applications of Agents
While we have explored a high-level overview of various agentic design patterns, grounding these concepts with real-world applications can help solidify our understanding of agent-based systems.
To achieve this, I will present case studies on agent implementations, drawing insights from practical case studies found in blogs from LangChain 16, LlamaIndex 17, and CrewAI 18. These case studies will showcase how different patterns are applied in real-world scenarios.
-
Captide – Financial Analysis 19:
- Core Business: Automating the extraction, integration, and analysis of data from investor relations documents.
- Key Tasks:
- Extracting financial metrics.
- Creating customized dataset.
- Generating contextual insights.
- Objective: To orchestrate a data retrieval and processing pipeline for a large corpus of financial documents.
- Patterns:
- Tool Use: Agents require access to a ticker-specific vector store to retrieve relevant financial data efficiently.
- Plan-and-Execute: Given the extensive volume of regulatory filings, parallelization is crucial for efficiency. The LLMCompiler pattern enables minimal latency while executing agent tasks concurrently.
- Reflection: To generate meaningful contextual insights from financial data, integrating the reflection pattern with tool use allows an iterative approach to refining the insights deliverable.
- Multi-agent: Specialized agents can enhance performance across key areas such as data extraction, data processing, and insights generation. By leveraging domain-specific expertise, these agents can improve accuracy, efficiency, and the overall quality of deliverables.
Considering potential regulatory compliance requirements, agents must adhere to financial regulations. A hallucinated “insider tip” or inaccurate insight could pose significant legal risks.
To mitigate such risks, integrating a human-in-the-loop approach – though not discussed in detail – can provide an additional layer of oversight. This ensures that all outputs align with legal and regulatory frameworks, safeguarding against compliance breaches.
-
Minimal – Customer Support 20:
- Core Business: Enhancing customer satisfaction by automating repetitive customer service workflows.
- Key Tasks:
- Providing context-rich responses to customer inquiries.
- Integrating with e-commerce platforms to perform actions (e.g., updating shipping addresses).
- Objective: To assist the support team in resolving complex Tier 2 and Tier 3 tickets — either as a copilot or in a fully autonomous capacity.
- Patterns:
-
Planning: Decomposes customer inquiries into sub-tasks, enabling specialized agents to retrieve task-specific information and follow appropriate protocols.
-
Tool Use: Leverages internal knowledge bases to retrieve relevant guidelines and information, enhancing the planning and accuracy of responses.
-
Multi-Agent: Integrates agents within a multi-agent system to facilitate final decisions, which may involve complex actions such as refunds or simpler tasks like providing responses.
Minimal adopted a multi-agent system approach after discovering that relying on a single large prompt for the LLM often conflated multiple tasks, leading to higher costs and increased errors.
-
-
- Core Business: Providing travel planning services with a focus on spontaneity and personalization.
- Key Tasks:
- Crafting hyper-personalized itineraries tailored to individual preferences.
- Adjusting plans dynamically based on real-time weather conditions and local updates.
- Objective: To deliver a unique travel experience for each customer through hyper-personalization.
- Patterns:
- Planning: Decomposes itinerary planning into sub-tasks based on customer preferences, travel dates, weather forecasts, and logistical considerations.
- Tool Use: Utilizes real-time weather updates to dynamically adjust plans and proactively inform users via messaging applications like WhatsApp.
- Multi-Agent: Agents collaborate to analyze customer preferences and determine the ideal travel destinations, continuously re-planning based on real-time location updates.
-
GymNation – Member Experience 22 23:
-
Core Business: Traditional gym operator and fitness community builder.
-
Key Tasks:
- Handling member inquiries.
- Appointment booking.
- Sales and prospecting outreach.
- Marketing and promotion.
-
Objective: To enhance the full lifecycle of a member’s experience through AI.
-
Patterns:
GymNation’s agent setup by Karl Foster 23 - Tool Use: Agents use tools to take decisive actions, such as booking appointments or retrieving information from sales sheets, to facilitate specialized workflows.
- Multi-Agent:
- The supervisor pattern is leveraged to route natural inputs, activating the agentic workflow and directing tasks to the most suitable agents.
- Given the extensive scope of a member’s lifecycle, the hierarchical pattern is also applied to further break down tasks, enabling more control and complexity for agents managing more involved processes.
-
While we’ve primarily explored case studies from leading LLM orchestration and agent frameworks, it is also valuable to examine more generalized agent case studies. These broader insights can provide a high-level perspective on key implementation decisions and patterns, helping to identify best practices and common challenges across different domains.
Building Your Own Agent: Lessons from Use-Cases
Having explored how existing businesses are leveraging agentic AI systems, it is crucial to distill key insights and best practices to guide the development of our own AI agents. By understanding the challenges, design patterns, and strategic decisions adopted by industry leaders, we can build more efficient, adaptable, and goal-oriented agents tailored to specific business needs.
-
Tool Design:
- Developing effective agentic AI systems requires prioritizing tools that align with the use case and operational needs. Key considerations include:
- Relevance and Functionality:
- If agent workflows require real-time data, tools must be designed to efficiently access APIs or databases for up-to-date information.
- Similar to RAG, if agents need to ground their responses in predefined business protocols or guidelines, tools that provide access to relevant databases are critical to ensure context-rich, accurate responses and minimize hallucinations.
- Security Measures:
- While tool usage is a fundamental pattern in agent design, robust security measures must be implemented to prevent unintended consequences.
- For example, in a report generation workflow where agents create charts using code, it’s essential to sandbox such tools (e.g., enforcing read-only access) to prevent unauthorized modifications and ensure system integrity.
- Relevance and Functionality:
- Developing effective agentic AI systems requires prioritizing tools that align with the use case and operational needs. Key considerations include:
-
Autonomy Levels:
- Designing agentic AI systems requires careful consideration of the level of autonomy granted to the agents.
Autonomy of Agents by LangGraph - Low Autonomy: Agents follow predefined workflows with fixed steps (e.g., structured data retrieval tasks managed via a router).
- Full Autonomy: Agents engage in open-ended problem-solving, with built-in fallbacks such as retry mechanisms and human escalation for critical decision points.
- Designing agentic AI systems requires careful consideration of the level of autonomy granted to the agents.
-
Evaluation:
- Building and deploying agentic AI workflows follow an iterative development process similar to traditional machine learning projects. Effective evaluation involves:
- Robust Testing:
- Agents should be tested with adversarial prompts to evaluate their behavior under potential misuse or rogue actors.
- Ensuring agents do not introduce risks, biases, or inaccuracies that could harm end-users is a critical component of the evaluation phase.
- Crawl-Walk-Run Approach:
- As highlighted in AI Engineering: Building Applications with Foundation 24, one way to evaluate AI applications (incld. agents) could follow a phased approach:
- Crawl: Initially, ensure mandatory human involvement in the AI workflow.
- Walk: Deploy the workflow internally for employee testing and validation.
- Run: Gradually increase automation and trust, allowing direct interaction with external users.
- As highlighted in AI Engineering: Building Applications with Foundation 24, one way to evaluate AI applications (incld. agents) could follow a phased approach:
- Demonstrating Business Value:
- While generative AI demonstrations and proof-of-concepts may appear impressive, the ultimate goal is to deliver tangible business value.
- Agents should contribute to measurable improvements, such as increased efficiency, cost savings, or enhanced customer satisfaction.
Closing Remarks
In this article, we’ve covered a range of topics – from defining agents to exploring key agentic design patterns and examining how enterprises are successfully leveraging agents in production environments.
However, it’s important to acknowledge that agents still face inherent challenges such as hallucinations, scalability issues, and ethical concerns (e.g., unchecked autonomy). While agents represent a significant step forward in integrating AI into the workforce and daily life, they still share many challenges with other AI applications we’ve encountered thus far.
Personally, I am excited about the future developments in the field of agentic AI, particularly:
- The emergence of smaller, specialized agents powered by distilled LLMs that are derived from larger, more capable models.
- Enhanced reasoning capabilities in advanced LLMs, such as OpenAI’s o1 and DeepSeek’s R1, which are promising to improve agent decision-making
Lastly, it’s inspiring to see the shift from traditional chatbots to intelligent problem-solving partners. As we move into the coming year, I look forward to spending more time understanding and designing thoughtful agent applications – and I hope you do too!
Further Learnings
- https://llmagents-learning.org/f24
- https://langchain-ai.github.io/langgraph/concepts/
- https://vitalflux.com/agentic-reasoning-design-patterns-in-ai-examples/
Footnotes
-
https://www2.deloitte.com/content/dam/Deloitte/us/Documents/consulting/us-state-of-gen-ai-q4.pdf ↩
-
https://www.anthropic.com/research/building-effective-agents ↩
-
https://blog.langchain.dev/how-captide-is-redefining-equity-research-with-agentic-workflows-built-on-langgraph-and-langsmith/ ↩
-
https://blog.langchain.dev/how-minimal-built-a-multi-agent-customer-support-system-with-langgraph-langsmith/ ↩
-
https://blog.crewai.com/how-waynabox-is-changing-travel-planning-with-crewai/ ↩
-
https://www.llamaindex.ai/blog/case-study-gymnation-revolutionizes-fitness-with-ai-agents-powering-member-experiences ↩
-
https://medium.com/@karl.foster/the-development-of-ai-agents-how-we-deployed-an-army-in-our-business-6c67f51e3d1d ↩ ↩2
-
https://www.amazon.sg/dp/1098166302?ref_=mr_referred_us_sg_sg ↩