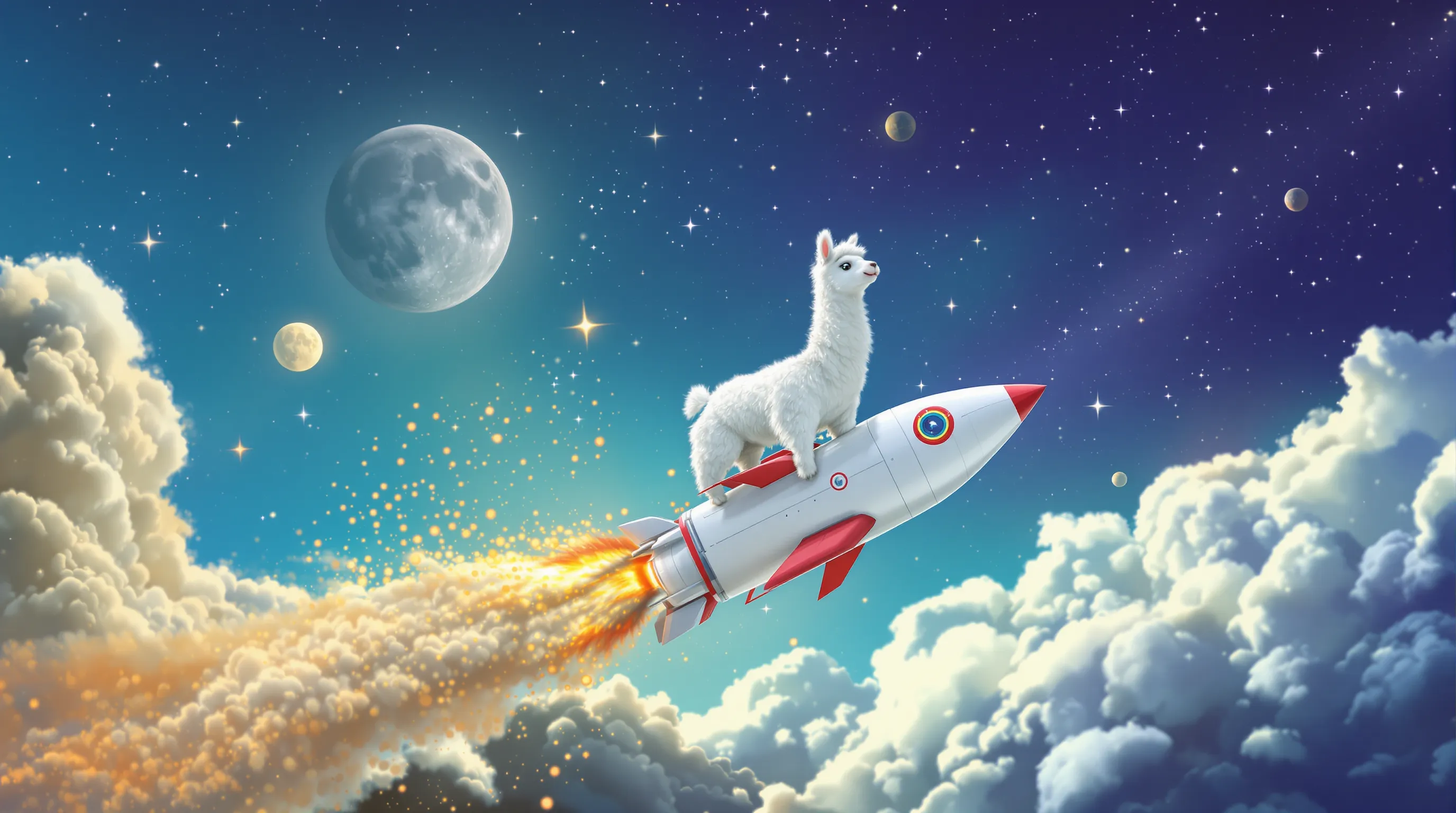
Exploring Speculative Decoding for LLM Inference
/ 23 min read
Introduction
This article extends on previously discussed LLM inference optimisations in my previous write-up.
As Large Language Models (LLMs) scale from 8 billion parameters (such as Meta-Llama-3.1-8B-Instruct
) to an astounding 405 billion parameters (like Meta-Llama-3.1-405B-Instruct
) and beyond for some closed-source models, the inefficiencies inherent in traditional auto-regressive decoding during inference become increasingly prohibitive.
Typically, transformers generate tokens sequentially, requiring a complete forward pass to produce each new token in a sequence 1. This approach results in significant inference latency, particularly as model size increases.
One effective strategy to address these inefficiencies is to optimize the token generation process through parallelization techniques. Speculative Decoding is one such approach, offering a promising solution to accelerate LLM inference.
Instead of generating tokens one by one, speculative decoding aims to produce multiple tokens in parallel. This method modifies the forward pass to generate several candidate tokens simultaneously. Afterward, each candidate is “verified” by the LLM responsible for the main task (e.g., text completion), where the model decides whether to accept or reject each candidate token.
In optimal conditions, speculative decoding can generate up to tokens in a single forward pass — a substantial improvement over traditional auto-regressive sampling, which generates only one token per pass. Even in the worst-case scenario, where all candidate tokens are rejected, the method still manages to generate a single token, maintaining comparable efficiency to standard auto-regressive decoding, albeit with additional overhead.
Without necessitating architectural modifications to the LLM, speculative decoding can typically be implemented through two main approaches:
- Speculative Sampling: Here, candidate tokens are generated in parallel using a draft model, and these candidates are then scored and selected by the target LLM.
- N-gram Decoding: In this approach, candidate tokens are generated based on n-grams derived from the input sequence and subsequently scored using the target model.
Existing research has shown that speculative decoding can yield a substantial speedup, achieving a 2-3x improvement over vanilla LLM decoding.
In this blog post, we’ll explore these speculative decoding techniques in greater detail, discussing how they can be implemented practically. We’ll also compare speculative decoding approaches to traditional auto-regressive sampling, evaluating their potential to substantially reduce inference latency.
Overview on Speculative Sampling with Draft Model
A great overview on Speculative Sampling with Draft Model by Efficient NLP.
To address the issues with auto-regressive sampling, Chen et al. (2023) proposed Speculative Sampling, an algorithm designed to accelerate LLM inference for latency-critical applications 2 3. The technique involves using a smaller, faster model, known as the draft model, alongside the primary target model.
Chen et al. (2023) proposed method is defined as the following algorithm in the paper:
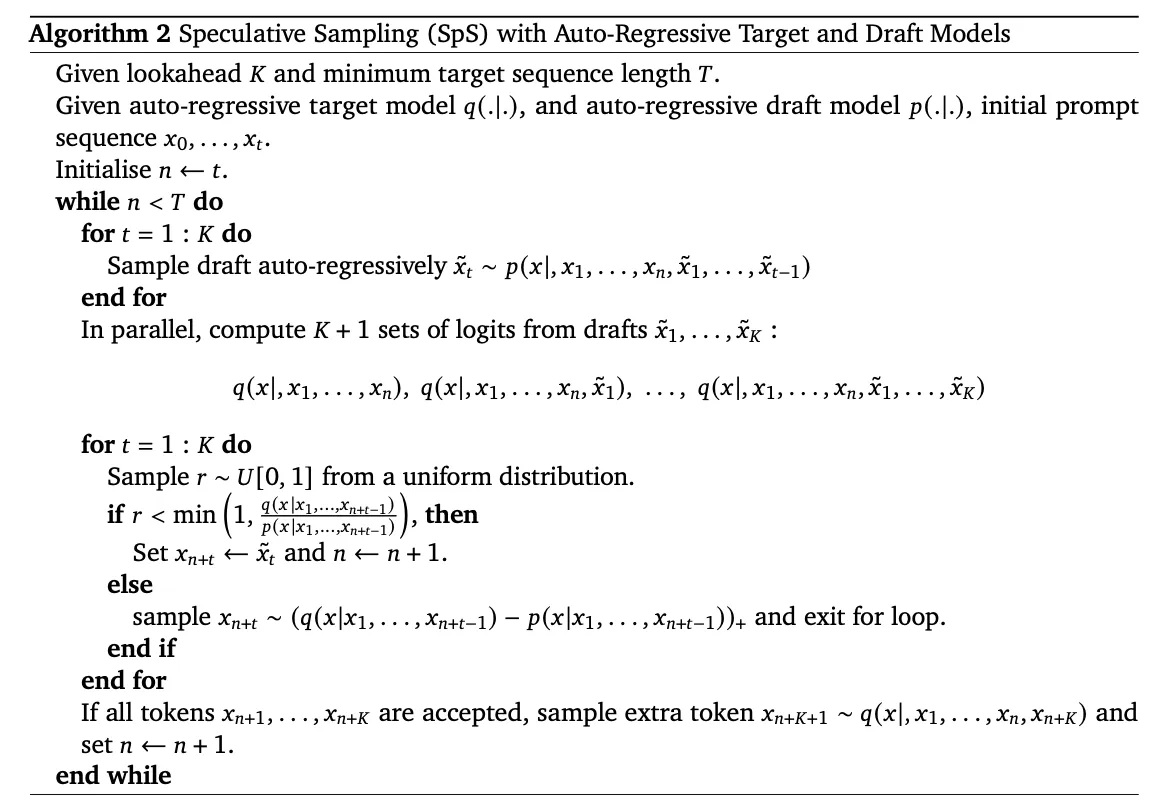
Algorithm of Speculative Sampling. Source: Chen et al. (2023).
This involves a target model – generally a large model that one is using for the main application e.g., Meta-Llama-3.1-70B-Instruct
and running a smaller (inherently faster) model, known as a draft model (e.g., Meta-Llama-3.1-8B-Instruct
) in parallel.
The draft model generates a sequence of candidate tokens auto-regressively, which are then scored by the target model. A modified rejection sampling method is employed to accept or reject these tokens based on the target model’s distribution. If any rejection occurs, we can resample token based on a recovered target model’s distribution.
The underlying intuition is that certain token sequences are “obvious,” where the distributions of both models align.
Since the draft model is typically much smaller, speculative sampling can generate candidate tokens more quickly than running the larger target model iteratively.
While the introduction of the draft model adds computational overhead, as long as the target model accepts a high proportion of draft tokens, this approach significantly reduces overall sampling latency without requiring modifications to the target model’s architecture.
Implementing Speculative Sampling
For full code implementation, refer to: https://github.com/wtlow003/speculative-sampling
To better understand speculative sampling, let’s implement the algorithm step-by-step following each core ideas from the paper.
Selection of Models
First, we need to define the pair of draft and target models. Chen et al. identified several strategies for selecting a draft model, but a straightforward approach is to choose a smaller version of the target model.
This selection ensures that both the draft and target models use the same tokenizer, guaranteeing that the sequences of tokens generated by the draft model align with those expected by the target model. With consistent tokenization, both models operate on the same fundamental representations of the text, enabling an accurate refinement process when accepting or rejecting the speculated tokens
For example, we can have the two models as:
- Draft Model:
Meta-Llama-3.1-8B
- Smaller and faster model used to speculate candidates tokens for scoring by target model
- Target Model:
Meta-Llama-3.1-70B
- Larger and slower model used for the main task we are trying to accomplish (e.g., text completion)
Speculative Sampling Loop
The outline of the speculative sampling algorithm is as follows:
- Draft models auto-regressively draft times
- Compute logits with the target model based on the drafted candidates
- Compare the draft probabilities with the target probabilities based on a modified rejection sampling scheme. If from the candidates is rejected based on the scoring, we stop accepting the candidates . Thereafter, we resample for , from a recovered target model’s distribution to obtain an accepted token.
- If all candidates is accepted, we can sample an additional token based on the logits produced by the target model previously computed based on the draft candidates.
Therefore, at each iteration of speculative sampling, we can generate 1 to tokens. This differs from auto-regressive sampling, where at each iteration, we are only generating 1 token.
The following is the implementation of the speculative sampling loop:
@torch.no_grad()def speculative_sampling( x: torch.Tensor, draft_model: torch.nn.Module, target_model: torch.nn.Module, N: int, K: int, temperature: float, top_k: int, top_p: float, eps: float = 1e-10,): """ Implementation of Algorithm 2 in the paper - Accelerating Large Language Model Decoding with Speculative Sampling (https://arxiv.org/abs/2302.01318). """ seq_len = x.shape[1] T = seq_len + N
# we will be increasing input x length until it reaches T while x.shape[1] < T: prefix = x x_len = x.shape[1]
# ----------------------------------------- # Step 1: Generate K tokens from draft_model # ----------------------------------------- generated_tokens = [] for _ in range(K): outputs = draft_model(prefix) p = outputs.logits next_token = sample( norm_logits(p[:, -1, :], temperature, top_k, top_p, eps) ) generated_tokens.append(next_token) prefix = torch.cat([prefix, next_token], dim=1)
generated_tokens = torch.cat(generated_tokens, dim=1) p = batch_norm_logits(p, temperature, top_k, top_p, eps) # type: ignore
# -------------------------------------------- # Step 2: Evaluate full sequence + K draft tokens using target_model # -------------------------------------------- q = target_model(prefix).logits q = batch_norm_logits(q, temperature, top_k, top_p, eps)
# ------------------------------ # Step 3: Single Round Rejection Sampling Process # ------------------------------ n = x_len - 1 target_probs = torch.gather( q[:, n : n + K, :], 2, generated_tokens.unsqueeze(-1) ).squeeze(-1) draft_probs = torch.gather( p[:, n : n + K, :], 2, generated_tokens.unsqueeze(-1) ).squeeze(-1)
# acceptance probabilities for all K tokens acceptance_probs = torch.minimum( torch.ones_like(target_probs), target_probs / draft_probs )
random_vals = torch.rand_like(acceptance_probs)
# determine which tokens are accepted accepted_tokens = random_vals < acceptance_probs
# ------------------------------ # Step 4: Combine Results and Resample if Necessary # ------------------------------ # determine where the first rejection occurs for each sequence first_rejection_indices = torch.nonzero(~accepted_tokens, as_tuple=True)[1]
if first_rejection_indices.all(): # if all tokens are accepted x = torch.cat([x, generated_tokens], dim=1) for token in generated_tokens[0]: yield token, True next_token = sample(q[:, -1, :]) yield next_token[0], True else: # if there is at least one rejection first_rejection_index = first_rejection_indices[0].item() selected_tokens = generated_tokens[:, :first_rejection_index] for token in selected_tokens[0]: yield token, True x = torch.cat([x, selected_tokens], dim=1) # recover probability distribution next_token = sample( max_fn( q[:, n + first_rejection_index, :] # type: ignore - p[:, n + first_rejection_index, :] # type: ignore ) ) yield next_token[0], False # print( # "rejected at", # n + first_rejection_index + 1, # " rejected token:", # generated_tokens[:, first_rejection_index], # type: ignore # " resampled token:", # next_token.squeeze(-1), # )
# add newly generated token to x x = torch.cat([x, next_token], dim=1)
return x
We will be going in-depth into different part of the loop to better understand how speculative sampling work.
Generate Candidates with Draft Model
Speculative sampling aims to accelerate the process of generating tokens by enabling parallel processing, thereby addressing the inefficiencies inherent in traditional auto-regressive token generation. The approach involves using a smaller, faster “draft” model to auto-regressively generate candidate tokens. These candidates can then be scored and used to produce the final output more efficiently.
The following is a simple for-loop to iteratively generate candidates:
# -----------------------------------------# Step 1: Generate K tokens from draft_model# -----------------------------------------generated_tokens = []for _ in range(K): outputs = draft_model(prefix) p = outputs.logits next_token = sample( norm_logits(p[:, -1, :], temperature, top_k, top_p, eps) ) generated_tokens.append(next_token) prefix = torch.cat([prefix, next_token], dim=1)
generated_tokens = torch.cat(generated_tokens, dim=1)p = batch_norm_logits(p, temperature, top_k, top_p, eps)
We apply adjustment and normalisation to the logits before sampling token, using the norm_logits()
function:
def top_k_p_filter(logits: torch.Tensor, top_k: int, top_p: float) -> torch.Tensor: top_k = min(top_k, logits.size(-1)) if top_k > 0: # # remove all tokens with probability less than the last token of top-k # indices_to_remove = logits < torch.topk(logits, top_k, dim=-1)[0][..., -1, None] # # set those filtered tokens to -inf # logits[indices_to_remove] = float("-inf") values, _ = torch.topk(logits, top_k) min_values = values[..., -1, None].expand_as(logits) logits = torch.where( logits < min_values, torch.full_like(logits, float("-inf")), logits ) if top_p > 0: sorted_logits, sorted_indices = torch.sort(logits, descending=True) cumulative_probs = torch.cumsum(F.softmax(sorted_logits, dim=-1), dim=-1) # determine indices to remove sorted_indices_to_remove = cumulative_probs > top_p # shift indices to the right to keep the same relative order sorted_indices_to_remove[..., 1:] = sorted_indices_to_remove[..., :-1].clone() # ensure we always keep top probability token even if it exceed cumulative probability sorted_indices_to_remove[..., 0] = 0 # # scatter sorted indices to remove to original indices # indices_to_remove = sorted_indices_to_remove.scatter( # dim=-1, index=sorted_indices, src=sorted_indices_to_remove # ) indices_to_remove = torch.zeros_like(logits, dtype=torch.bool).scatter_( dim=-1, index=sorted_indices, src=sorted_indices_to_remove ) # # set logits of the indices to remove to -inf # logits[indices_to_remove] = float("-inf") logits = logits.masked_fill(indices_to_remove, float("-inf")) return logits
def norm_logits( logits: torch.Tensor, temperature: float, top_k: int, top_p: float, eps: float) -> torch.Tensor: """ Normalize logits by temperature and top-k/p filters. """ # logits – shape: [batch_size, vocab_size] assert logits.dim() == 2 # temperature scaling: control "softness" or "sharpness" of probability distribution generated by softmax logits = logits / (temperature + eps) # [batch_size, vocab_size] logits = top_k_p_filter(logits, top_k, top_p) # generate probability distribution return F.softmax(logits, dim=-1)
- In particular, we applied
temperature scaling
andtop-k/p
filters to the logits before converting them into probabilities.
After we generated candidates, we batch normalize the logits from the last auto-regressive sampling iteration to convert the logits into probabilities from the draft model ():
def batch_norm_logits( logits: torch.Tensor, temperature: float, top_k: int, top_p: float, eps: float) -> torch.Tensor: # assuming logits shape is [batch_size, sequence_length, vocab_size] batch_size, seq_len, vocab_size = logits.shape logits = logits.view(-1, vocab_size) probs = norm_logits(logits, temperature, top_k, top_p, eps) return probs.view(batch_size, seq_len, vocab_size)
Computing Logits with Target Model
Given the candidates generated, we concatenated the sampled candidate tokens with the existing sequence and computed the respective logits at each position with the target model, resulting in logits. Subsequently, we also batch normalize the logits to produce the target model probabilities ():
# --------------------------------------------# Step 2: Evaluate full sequence + K draft tokens using target_model# --------------------------------------------q = target_model(prefix).logitsq = batch_norm_logits(q, temperature, top_k, top_p, eps)
Modified Rejection Sampling Scheme
With both the draft model probabilities () and target model probabilities (), computed, we can begin to score to accept or reject the draft candidates .
We begin by extracting out the probability of each candidate token from both the draft and target model probabilities.
n = x_len - 1
target_probs = torch.gather( q[:, n : n + K, :], 2, generated_tokens.unsqueeze(-1)).squeeze(-1)
draft_probs = torch.gather( p[:, n : n + K, :], 2, generated_tokens.unsqueeze(-1)).squeeze(-1)
x_len
refers to the length ofx
(input_ids) at the current iteration
Subsequently, we compare and determine which candidate token is accepted or rejected and identify the index where the first rejection occurs:
# acceptance probabilities for all K tokensacceptance_probs = torch.minimum( torch.ones_like(target_probs), target_probs / draft_probs)
random_vals = torch.rand_like(acceptance_probs)
# determine which tokens are acceptedaccepted_tokens = random_vals < acceptance_probs
# determine where the first rejection occurs for each sequencefirst_rejection_indices = torch.nonzero(~accepted_tokens, as_tuple=True)[1]
In the case, where all candidates are accepted, we can also further sample an addition token from the logit as previously computed by the target model:
if first_rejection_indices.all(): # if all tokens are accepted x = torch.cat([x, generated_tokens], dim=1) next_token = sample(q[:, -1, :])else: ...
However, if we noticed that rejection did occur, we will choose the candidate tokens up to the first rejected candidate . We accept all candidate tokens up to the first rejected candidate, .
Meanwhile for the rejected , we will resample from a recovered target distribution. For mathematical proof on how speculative sampling’s rejection sampling scheme can recover the target model’s distribution for accepted tokens, please refer to Theorem 1.
if first_rejection_indices.all(): ...else: # if there is at least one rejection first_rejection_index = first_rejection_indices[0].item() x = torch.cat([x, generated_tokens[:, :first_rejection_index]], dim=1) # recover probability distribution next_token = sample( max_fn( q[:, n + first_rejection_index, :] # type: ignore - p[:, n + first_rejection_index, :] # type: ignore ) )
Regardless whether full acceptance or rejection occurs during speculative sampling, a new token will be determined (through logit or resampling for rejected ). This new token should be concatenated to the existing sequence, preparing the sequence for the next iteration of the speculative sampling loop. This process continues iteratively until the maximum output token length, defined as , is reached.
# add newly generated token to xx = torch.cat([x, next_token], dim=1)
Overview on N-gram Decoding
In both LLMA Decoding (Yang et al., 2023) 4 and Prompt Lookup Decoding (Saxena, 2023) 5, the primary goal is to accelerate LLM inference through a shared core idea: leveraging n-grams to identify candidate tokens in each iteration of the LLM forward pass.
This idea is rooted in the observation that many LLM use-cases involving input-grounded generation — such as summarization, code editing, and multi-turn chat — often include identical text spans between the LLM’s output and the reference input prompt. This overlap suggests that high n-grams similarity occurs as the LLM “copies” from the input prompt and incorporates it into the output.
To capitalize on this behavior, n-gram decoding (as named in the implementations in transformers
/vLLM
) searches for sub-sequences within the prefix formed by existing tokens to match text spans found in the input prompt.
When a matching prefix is identified based on the n-grams, the method then selects up to candidate tokens from the subsequent sequence of text that follows the matched span.
Unlike Speculative Sampling, n-gram decoding does not require a draft model.
Although, Saxena (2023) introduced prompt lookup decoding, which was later implemented in the transformers
library.
The original work did not include a written algorithm. Thankfully, Yang et al. (2023) provided a clear algorithm for LLMA decoding, which is detailed as follows:
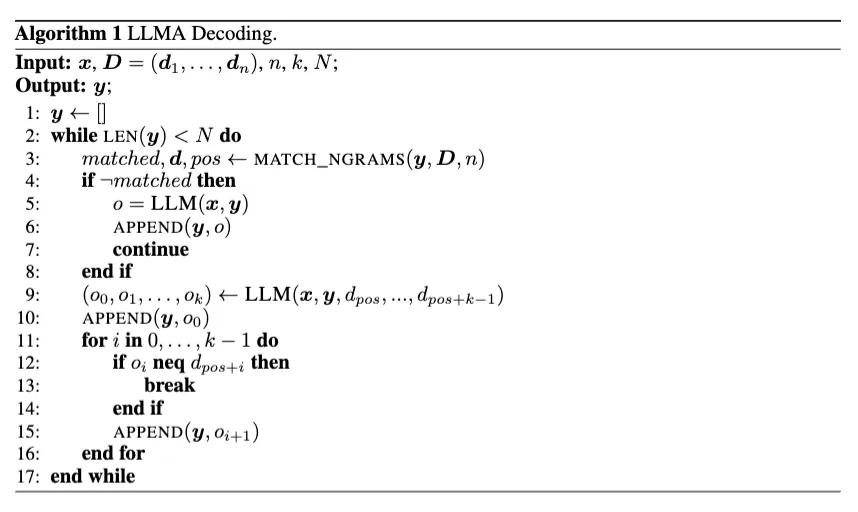
Algorithm of LLMA Decoding. Source: Yang et al. (2023).
However, based on the implementation example provided by Saxena, we noticed overlapping concepts between the implementations of both proposed decoding methods. Given these similarities, we will integrate key ideas from both Saxena’s and Yang et al.’s work into a unified n-grams decoding approach in the following section.
Implementing N-gram Decoding
For full code implementation, refer to: wtlow003/ngram-decoding
To better understand n-gram decoding, let’s implement the algorithm step by step, following the core ideas from the implementations we discussed earlier.
N-gram Decoding Loop
The outline of the n-gram decoding algorithm is as follows:
- Extract an -length prefix from the end of the input token sequence and match the prefix against the input token sequence to identify potential matches.
- For each identified match, determine the candidate tokens that follow the matched prefix. Among the options, select the sequence with the longest matched prefix and candidate tokens; if multiple sequences have the longest match, break ties by random selection. If no matches or candidates are identified, revert to single-step auto-regressive decoding.
- Compute the logits for the tokens using the LLM, processing the candidate tokens in parallel.
- Compare the generated tokens with the candidates to identify exact matches. For any mismatch at position , restrict the selection from the set and select up to valid candidates accordingly.
- If all candidates are validated, decode an additional token based on the logits corresponding to the position previously computed in (3).
Therefore, similar to speculative sampling, at each iteration, n-gram decoding allow us to generate 1 to tokens.
The following is the entire n-gram decoding loop:
@torch.no_grad()def ngram_decoding( input_ids: torch.Tensor, model: torch.nn.Module, tokenizer: Union[PreTrainedTokenizer, PreTrainedTokenizerFast], ngrams_size: int, K: int, n: int,): eos_token_id = tokenizer.eos_token_id if tokenizer.eos_token_id is not None else 0 eos_token_id_tensor = torch.tensor( [eos_token_id], dtype=torch.long, device=input_ids.device ) seq_len = input_ids.shape[1] T = seq_len + n
while input_ids.shape[1] < T: prefix = input_ids cur_len = input_ids.shape[1]
# ----------------------------------------- # Step 1: Generate N-grams # -----------------------------------------
n_grams = input_ids[0, -ngrams_size:]
# ----------------------------------------- # Step 2: Generate K candidates tokens using the N-grams # -----------------------------------------
candidate_tokens = generate_candidate_tokens(input_ids, n_grams, ngrams_size, K)
# ----------------------------------------- # Step 3: Validate the candidates using the LLM # -----------------------------------------
# based on: https://arxiv.org/pdf/2304.04487 # if we did not find any candidates tokens, we default to single-step decoding if candidate_tokens.shape[1] == 0: logits = model(input_ids).logits[:, -1, :] next_token = logits.argmax(dim=-1) input_ids = torch.cat([input_ids, next_token.unsqueeze(dim=0)], dim=1) yield (next_token.item(), False) if next_token.item() == eos_token_id: break continue
prefix = torch.cat([input_ids, candidate_tokens], dim=1) # include the max(1, K) + 1 in the logits logits = model(prefix).logits[:, -candidate_tokens.shape[1] - 1 :, :]
assert ( logits.shape[1] == candidate_tokens.shape[1] + 1 ), f"Expected logits shape: {candidate_tokens.shape[1] + 1}, got: {logits.shape[1]}"
selected_tokens = logits.argmax(dim=-1) # calculate the number of consecutive matching tokens between candidate_tokens and selected_tokens n_matches = ( (~(candidate_tokens == selected_tokens[:, :-1])).cumsum(dim=-1) < 1 ).sum() # minus 1 to prevent overshooting max allowable tokens n_matches = min(n_matches, T - cur_len - 1)
# this allow us to generate at least 1 token or K+1 tokens max valid_tokens = selected_tokens[:, : n_matches + 1] # print("selected from prompt: ", tokenizer.decode(valid_tokens[0])) for token_id in valid_tokens[0]: yield (token_id.item(), True) input_ids = torch.cat([input_ids, valid_tokens], dim=1)
if input_ids.shape[1] >= T: # Check if we've reached the desired length break
if (valid_tokens == eos_token_id_tensor.item()).any(): break
return input_ids
We will be going in-depth into different part of the loop to better understand how n-gram decoding works.
Create N-gram Prefix
To form the n-gram prefix for matching in the input prompt, we take the last ngrams_size
tokens from the current input sequence at each iteration:
# -----------------------------------------# Step 1: Generate N-grams# -----------------------------------------
n_grams = input_ids[0, -ngrams_size:]
Generate Candidates with N-gram Prefix
To generate candidate tokens, we need to match the derived n-gram with the current input sequence:
# -----------------------------------------# Step 2: Generate K candidates tokens using the N-grams# -----------------------------------------
candidate_tokens = generate_candidate_tokens(input_ids, n_grams, ngrams_size, K)
This is done via a helper function – generate_candidate_tokens()
:
# adapted from: https://github.com/apoorvumang/prompt-lookup-decoding?tab=readme-ov-filedef generate_candidate_tokens( input_ids: torch.Tensor, n_grams: torch.Tensor, ngrams_size: int, K: int): # unfold the tensor into windows of `pattern_len + following_elements_count` window = input_ids.unfold(dimension=1, size=ngrams_size, step=1) # compare each window with the pattern (only the parts corresponding to the pattern) matching_window_indices = (window == n_grams).all(dim=2) # extract the indices where there are matches matching_indices = matching_window_indices.nonzero(as_tuple=True)[1]
# find candidates with the longest length # based on: https://arxiv.org/pdf/2304.04487 # we choose the candidate with the longest length at random if there are multiple candidates candidates = [] min_length = 1 for idx in matching_indices: start_idx = idx + ngrams_size end_idx = start_idx + K candidate = input_ids[0, start_idx : min(end_idx, input_ids.size(1))] length = len(candidate)
if length == min_length: candidates.append(candidate) elif length > min_length: min_length = length candidates = [candidate]
if candidates: chosen_candidate = candidates[np.random.randint(len(candidates))] else: chosen_candidate = torch.tensor([], dtype=torch.long, device=input_ids.device)
return chosen_candidate.unsqueeze(dim=0)
- Majority of the code is derived from Saxena’s implementation. However, I incorporated ideas from Yang et al., by e.g, tie-breaking same length candidates at random.
Defaulting to Single-Step Greedy Decoding
If no candidate tokens are generated from our attempt in matching our n-gram prefix, we will seek to default to single-step greedy decoding as described in LLMA decoding and finish the current iteration early:
# based on: https://arxiv.org/pdf/2304.04487# if we did not find any candidates tokens, we default to single-step decodingif candidate_tokens.shape[1] == 0: logits = model(input_ids).logits[:, -1, :] next_token = logits.argmax(dim=-1) input_ids = torch.cat([input_ids, next_token.unsqueeze(dim=0)], dim=1) yield (next_token.item(), False) if next_token.item() == eos_token_id: break continue
Computing Logits with Model
If we have at least 1 candidate token , we will proceed to generate the max(1, K+1) + 1
logits with the LLM:
prefix = torch.cat([input_ids, candidate_tokens], dim=1)# include the max(1, K) + 1 in the logitslogits = model(prefix).logits[:, -candidate_tokens.shape[1] - 1 :, :]
assert ( logits.shape[1] == candidate_tokens.shape[1] + 1), f"Expected logits shape: {candidate_tokens.shape[1] + 1}, got: {logits.shape[1]}"
Comparing Candidate and Actual Tokens
Once we have computed the logits from the LLM, we can conduct greedy decoding and identify the exact matches between the candidate and actual tokens. The objective is to identify the number of matches up to the first mismatched token.
In the event all candidate tokens are accepted, we can naturally decode the token as computed from the logits by the LLM:
selected_tokens = logits.argmax(dim=-1)
# calculate the number of consecutive matching tokens between candidate_tokens and selected_tokensn_matches = ( (~(candidate_tokens == selected_tokens[:, :-1])).cumsum(dim=-1) < 1).sum()
# minus 1 to prevent overshooting max allowable tokensn_matches = min(n_matches, T - cur_len - 1)
# this allow us to generate at least 1 token or K+1 tokens maxvalid_tokens = selected_tokens[:, : n_matches + 1]
Results
In this section, we will conduct a preliminary comparison of n-gram decoding and speculative sampling performance. Our goal is to gain a quick insight into the effectiveness of these speculative decoding methods in reducing inference latency.
While the comparisons may not be exhaustive, it should provide a useful initial assessment of the potential benefits of these speculative decoding strategies.
Speculative Sampling
For speculative sampling, I compared the inference latency of the model with vanilla auto-regressive sampling vs. speculative sampling.
The following command was used to run the experiment based on the following script:
# auto-regressive Samplingpython main.py --target-model unsloth/Meta-Llama-3.1-70B-bnb-4bit \ --sampling-method autoregressive \ --input-str "Alan Turing theorized that computers would one day become" \ --N 50 \ --temperature 0 \ --top-k 0 \ --top-p 0
# speculative samplingpython main.py --target-model unsloth/Meta-Llama-3.1-70B-bnb-4bit \ --draft-model unsloth/Meta-Llama-3.1-8B-bnb-4bit \ --sampling-method speculative \ --input-str "Alan Turing theorized that computers would one day become" \ --N 50 \ --K 4 \ --temperature 0 \ --top-k 0 \ --top-p 0
The following video shows the inference latency of both sampling methods:
The final results are as follows:
Method | Time | Token/sec | Speedup |
---|---|---|---|
Autoregressive Sampling | 27.7 | 1.79 | 1.00 |
Speculative Sampling | 11.3 | 4.68 | ~2.61x |
When compared to other prior write-ups and implementations of speculative sampling, such as the one detailed here, we observed a comparable level of performance, achieving approximately a ~2x speedup with speculative sampling.
- Note: Unlike other implementations, I did not perform an extensive benchmark to conclusively determine potential speedup across different scenarios e.g, varying model size, number of tokens, etc.
- However, I have linked other implementations of speculative sampling in the references section, where you can take a look at results from more detailed benchmarkings.
In Chen et al. (2023), they observed substantial speed up of up to ~2.5x when experiment based on a Chinchilla 70B and 7B target and draft model setup
N-gram Decoding
For n-gram decoding, I compared the model’s inference latency using vanilla greedy decoding versus n-gram decoding. Based on the underlying concept of n-gram decoding, the inference latency should be reduced in tasks involving input-grounded generation.
In this case, I used a prompt that involves the task of code-editing, where there should be many repeated text spans between the input prompt and the LLM’s output:
<|start_header_id|>user<|end_header_id|>
Code: def generate_candidate_tokens( input_ids: torch.Tensor, n_grams: torch.Tensor, ngrams_size: int, K: int ): # unfold the tensor into windows of `pattern_len + following_elements_count` window = input_ids.unfold(dimension=1, size=ngrams_size, step=1) # compare each window with the pattern (only the parts corresponding to the pattern) matching_window_indices = (window == n_grams).all(dim=2) # extract the indices where there are matches matching_indices = matching_window_indices.nonzero(as_tuple=True)[1]
# find candidates with the longest length # based on: https://arxiv.org/pdf/2304.04487 # we choose the candidate with the longest length at random if there are multiple candidates candidates = [] max_length = K for idx in matching_indices: start_idx = idx + ngrams_size end_idx = start_idx + K candidate = input_ids[0, start_idx : min(end_idx, input_ids.size(1))] length = len(candidate)
if length == max_length: candidates.append(candidate) else: # we do not consider prefix with no candidates if length > max_length: max_length = length candidates = [candidate]
if candidates: chosen_candidate = candidates[np.random.randint(len(candidates))] else: chosen_candidate = torch.tensor([], dtype=torch.long, device=input_ids.device)
return chosen_candidate.unsqueeze(dim=0)
Question: Can you the variable name 'candidates' to 'candidates_tokens'?
Modified code:<|start_header_id|>assistant<|end_header_id|>
The following command was used to run the experiment based on the following script:
# ngram decodingpython main.py --model meta-llama/Meta-Llama-3.1-8B-Instruct \ --decoding-method ngram
# greedy decodingpython main.py --model meta-llama/Meta-Llama-3.1-8B-Instruct \ --decoding-method greedy
The following video shows the inference latency of both decoding methods:
The final results are as follows:
Decoding Method | Time Taken (s) | Tokens/sec | Speedup |
---|---|---|---|
Greedy Decoding | 26.4 | 14.0 | 1x |
Ngrams Decoding | 12.8 | 28.9 | ~2x |
In the simple demonstration experiment, we achieved results comparable to those of the original prompt lookup decoding implementation and the figures reported in LLMA decoding. Both speculative decoding methods demonstrated approximately a 2-3x improvement in speed over greedy decoding.
Conclusion
To recap, we have discussed two popular speculative decoding methods: speculative sampling and n-gram decoding. We looked into the core ideas of both methods and attempted to implement them.
I also demonstrated in a non-conclusive manner through simple experiments that speculative decoding methods can be effective in reducing inference latency, with potential ~2x speedup over vanilla LLM decoding methods.
However, is important to note that speculative decoding methods explored are not exhaustive. The methods explored in this article are speculative decoding methods that do not require architecture changes to the underlying LLM models.
There are other methods such as MEDUSA 6 and EAGLE 7 8 that requires re-training the model with slight architecture changes for faster decoding. In the near future, I will be looking into these methods and provide a more detailed comparison.
In the case of EAGLE, it is said to be evaluated as the fastest speculative decoding method based on Spec-Bench!
I hope you learn something new, and till next time!
References
- https://research.ibm.com/blog/speculative-decoding
- https://www.youtube.com/watch?v=S-8yr_RibJ4
- https://philkrav.com/posts/speculative/
- https://towardsdatascience.com/speculative-sampling-intuitively-and-exhaustively-explained-2daca347dbb9
- https://github.com/openai/gpt-2/issues/209
- https://github.com/apoorvumang/prompt-lookup-decoding
- https://github.com/microsoft/LMOps/tree/main/llma
- https://github.com/feifeibear/LLMSpeculativeSampling
- https://github.com/jaymody/speculative-sampling
- https://gist.github.com/bsantraigi/5752667525d88d375207f099bd78818b
- https://medium.com/ai-science/speculative-decoding-make-llm-inference-faster-c004501af120
- https://jaykmody.com/blog/speculative-sampling/
Footnotes
-
To better understand how LLM inference works and existing decoding strategies, refer to the following Hugging Face blog post. ↩
-
Chen, M., et al. (2023). Speculative Sampling for Accelerating Large Language Model Inference. arXiv preprint arXiv:2302.01318. ↩
-
Around the same time, Google also released a similar paper: Leviathan, Y., Kalman, M., & Matias, Y. (2023). Fast Inference from Transformers via Speculative Decoding. arXiv preprint arXiv:2211.17192. ↩
-
Yang, L., et al. (2023). Inference with Reference: Lossless Acceleration of Large Language Model. arXiv preprint arXiv:2304.04487. ↩
-
Saxena, A. (2023). Prompt Lookup Decoding. GitHub repository. ↩
-
Cai, T., et al. (2024). MEDUSA: Simple LLM Inference Acceleration Framework with Multiple Decoding Heads. arXiv preprint arXiv:2401.10774. ↩
-
Li, Y., et al. (2024). EAGLE: Speculative Sampling Requires Rethinking Feature Uncertainty. arXiv preprint arXiv:2401.15077. ↩
-
Li, Y., et al. (2024). EAGLE-2: Faster Inference of Language Models with Dynamic Draft Trees. arXiv preprint arXiv:2406.16858. ↩