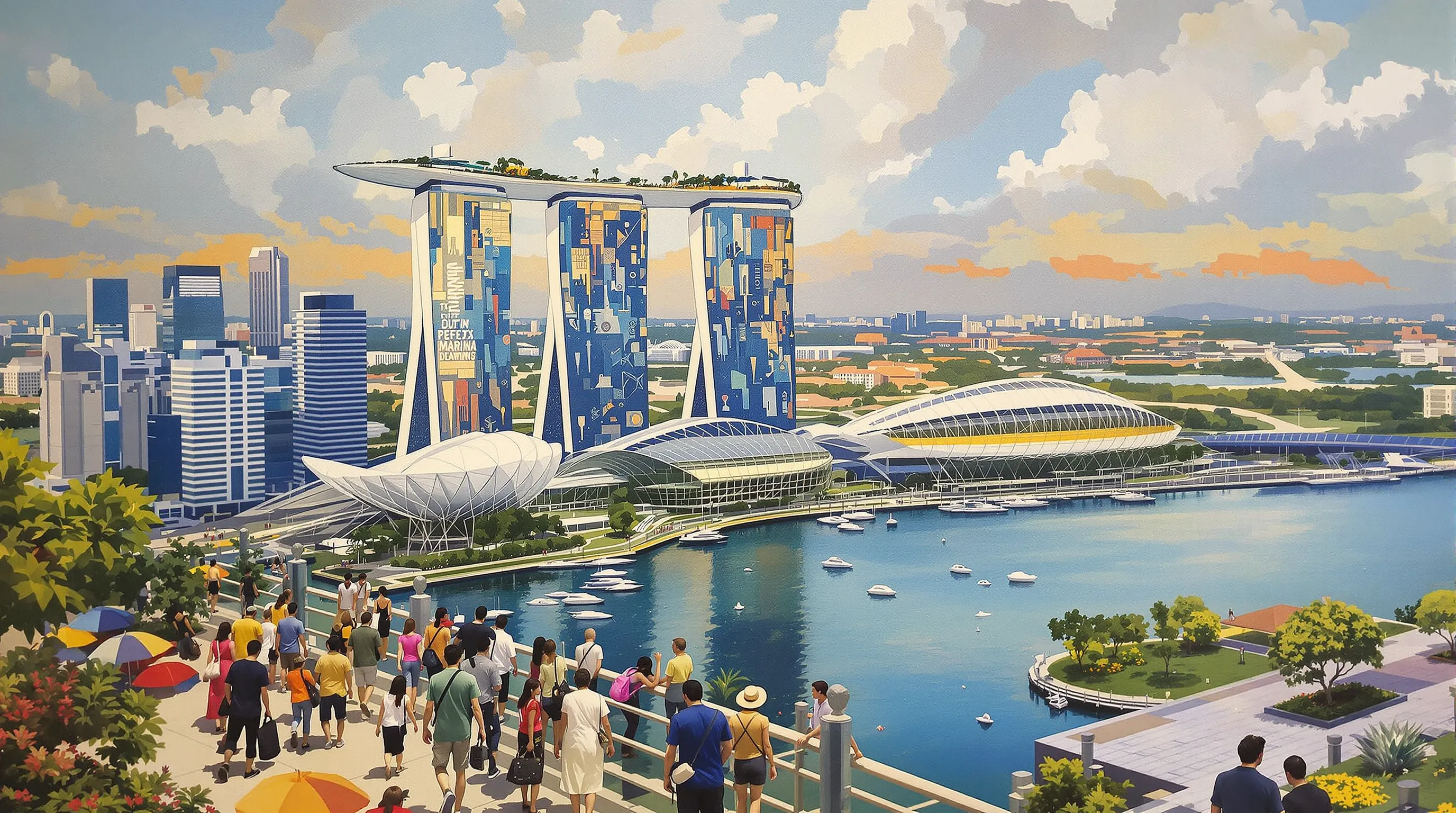
Singlish-Whisper: Finetuning ASR for Singapore's Unique English
/ 28 min read
Introduction
By now, many of you have likely heard of Whisper, an open-source Automatic Speech Recognition (ASR
) model developed by OpenAI.
It is currently regarded as one of the best models on the market.
I have personally used it for several projects, including video2article, to transcribe YouTube videos automatically.
This saved me a significant amount of time and effort compared to doing it manually.
Whisper is based on the traditional encoder-decoder Transformers architecture. It differs from previous ASR models such as wav2vec in its training approach. Instead of being self-supervised, Whisper is trained on a large, diverse, multi-lingual dataset consisting of 680,000 hours of audio collected from the web. Thanks to the diversity in its dataset, with more than one-third of the data coming from non-English languages, Whisper is able to generalize better to different languages. Additionally, it excels in tasks that require translation.
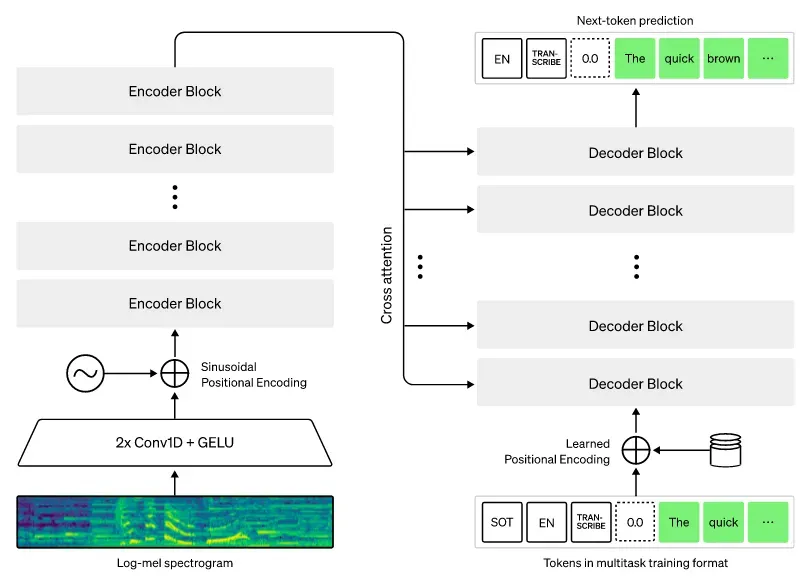
Architecture of Whisper models. Source: OpenAI.
While Whisper is known to perform decently on several languages, it still struggles with low-resource languages. I was wondering if Singlish is one of these languages.
Although not a formal language, Singlish, or Singapore English, is an informal, colloquial form of English unique to Singapore. It reflects the country’s multi-cultural diversity within the language itself.
As a Singaporean, I have been using Singlish for most of my life. When talking to my foreign friends, they often find it difficult to understand. This led me to a question:
Can one of the best ASR models understand Singlish?
If not, can we make it better?
In this blog, I will explore the possibility of fine-tuning Whisper to better process English-based creole languages like Singlish. The focus will be on fine-tuning smaller Whisper models, such as whisper-small, to better handle Singlish in a more cost-efficient way.
Generally, larger models perform better. With the largest and best Whisper model, like whisper-large-v3, the performance with Singlish is already impressive.
- You can skip to this section, where you can see the out-of-the-box performance of whisper-large-v3 with Singlish.
Understanding Singlish
If you are Singaporean, Singlish should be no stranger to you. It is likely already part of your daily spoken language, ingrained from a young age like many others. Singlish — Singaporean English — is a local form of spoken English that is a melting pot of languages and dialects, reflecting Singapore’s multi-cultural and multi-ethnic background.
The deeply integrated multi-cultural society of Singapore means that speakers of various languages and dialects often come into contact. Languages such as Bahasa Melayu and dialects like Teochew and Hokkien have mixed over the years to form the language we know today as Singlish.
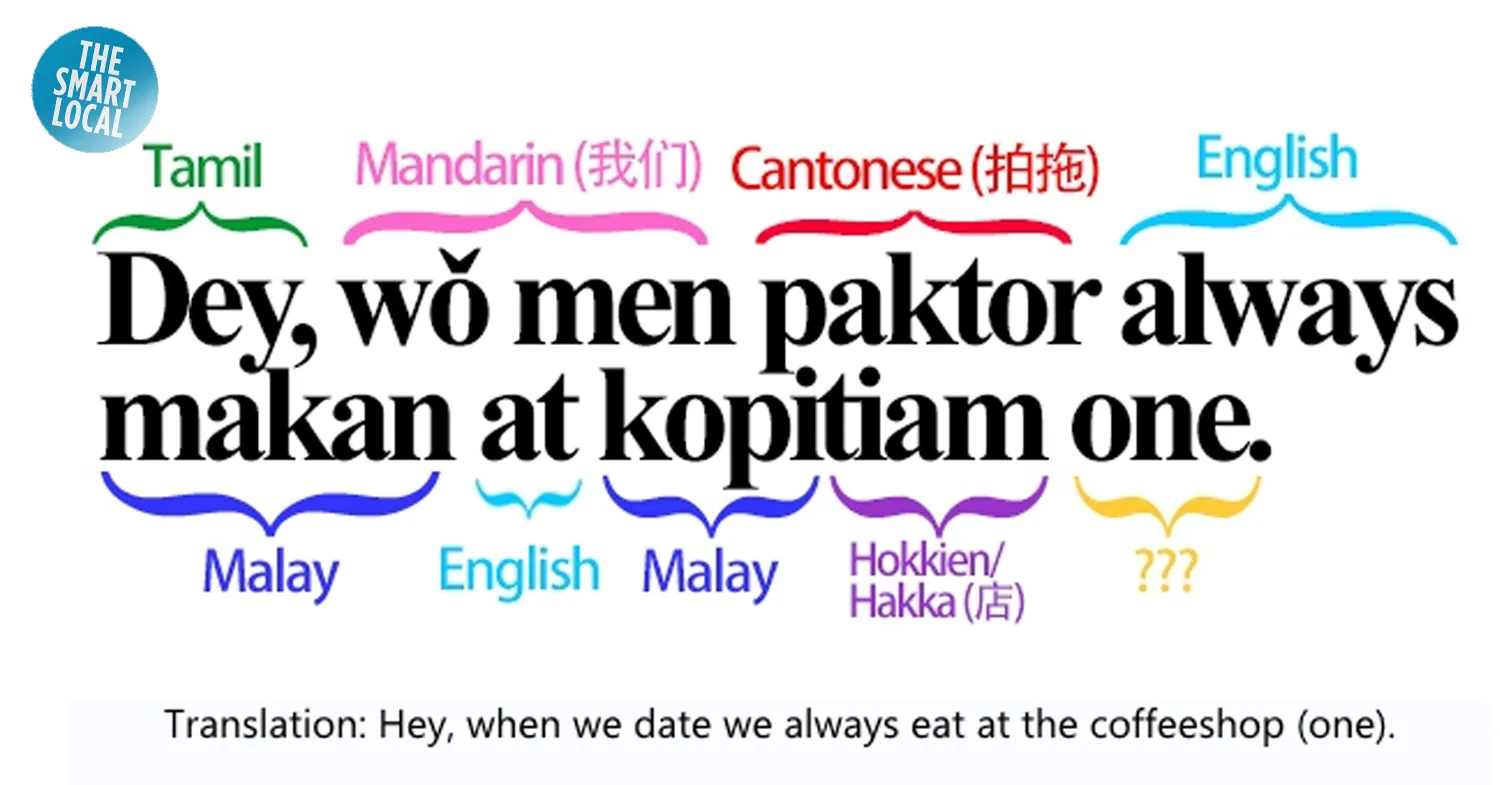
Example of Singlish sentence structure. Source: Renae Cheng.
While Singlish is something truly unique to Singapore, it faces potential challenges when it comes to the latest technology. Singlish, as the name implies, is based on English but includes a mix of other languages, dialects, and slang. Its overall meaning may not be easily understood by ASR models, such as Whisper, which are trained on more generic forms of English datasets. These models may not account for the nuances of a local language like Singlish.
For example, consider the following sentence:
I am meeting my friends at Jalan Kayu for prata today.
While the whole sentence is predominantly in English, the model might struggle to understand the pronunciation of non-English words like Jalan Kayu
and prata
that are mixed into the sentence.
- Jalan Kayu: Originates from the Malay language, where “jalan” means “street” and “kayu” means “wood.”
- Prata: Originates from the Sanskrit, where the word “paratha” generally means “layers of cooked dough.”
Getting the Data: IMDA’s National Speech Corpus
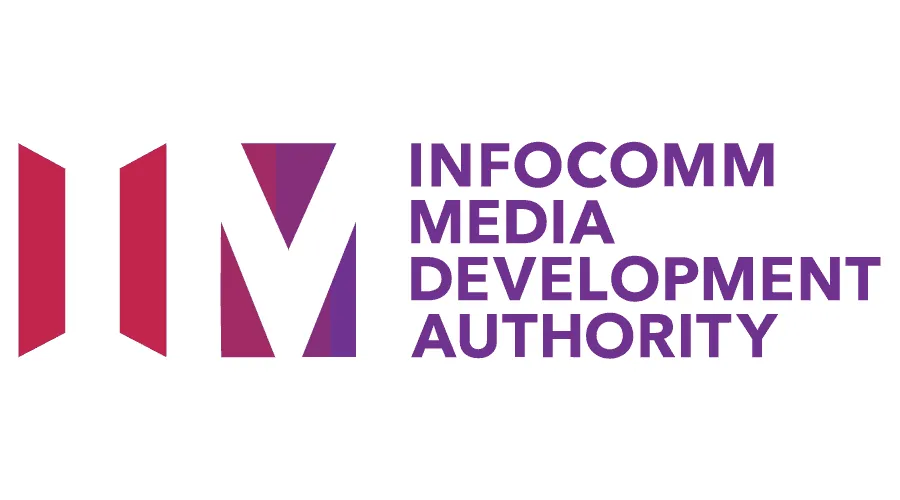
To fine-tune Whisper for Singlish, we need a dataset of audio samples that are representative of the local slang and accent.
Fortunately, the Info-communications and Media Development Authority (IMDA
) has developed the National Speech Corpus (NSC
), which is the first large-scale Singapore English corpus.
If you are interested in obtaining the dataset, you can fill in your information using the following link.
Recognizing the increasing use of voice interaction services in daily life and the limitations of current speech technologies in handling locally accented English, the corpus aims to bridge this gap. It provides a large dataset of audio samples and transcripts to improve ASR models for Singlish.
As of the last update in July 2021, the NSC corpus is approximately 1.2 TB in size, divided into six different parts. Each part includes audio samples taken in various scenarios. In some of the earlier parts, the audio samples consist of prompted recordings of participants reading scripts or random sentences involving local words and entities. In the later parts, the audio samples include participants conversing about daily life, engaging in debates, or recordings from call center settings.
In our effort to fine-tune Whisper for Singlish, we will use the sections containing prompted recordings of random sentences that include local words, names, and entities. This selection is intentional because these parts are more representative of the local slang and accent, allowing us to cover more words and phrases unique to Singapore.
While the latter parts of the dataset may be more suitable for fine-tuning ASR models to handle general conversations, our project’s objective is to explore the possibility of improving existing smaller models like whisper-small to better process English-based creole languages, such as Singlish, without focusing on specific scenarios.
The specific part in the NSC is labeled as PART2
in the Dropbox link.
To better understand the dataset, I have provided samples that include local words and entities such as street names, individual names, food items and brands. These audio samples feature individuals from a diverse range of ethnicities and genders, providing a comprehensive representation of the local accents associated with each group.
"Tay Kay Chin, Wong Kan Seng and Ee Peng Liang? Are you not familiar with these names?"
The audio samples provided are not part of our eventual training dataset.
As you may have noticed in the provided audio samples, even Singaporeans occasionally mispronounce local terms. This is often due to patterned errors, such as Malay or Indian speakers mispronouncing certain sounds in Chinese names, or participants encountering unfamiliar words or phrases.
Despite these occasional inaccuracies, the audio samples still offer a diverse representation of how typical Singaporeans pronounce various words and phrases.
Baseline Performance
While the prompted speech portion of the NSC contains approximately 1,000 hours of read speech data, it would be impractical to fine-tune Whisper on the entire dataset due to my limited computational resources.
It’s important to note that since we are only using a subset of the data, we may not fully capture the entire diversity of the local language.
Therefore, I have extracted subsets of the data for fine-tuning Whisper. Furthermore, to better understand how the amount of data affects the model’s performance, I have chosen to fine-tune Whisper on two different subsets of varying sizes, selected based on reasonable criteria.
In addition to selecting a subset of the data, I have chosen the whisper-small model for fine-tuning due to its smaller size and faster inference speed.
The following provides detailed information about the selected subset(s):
Subset | Total Samples | Total Hours | Speakers | Split (train/valid/test) |
---|---|---|---|---|
1 | 39,661 | ~52 hours | 57 | 80/10/10 |
2 | 122,142 | ~163 hours | 161 | 80/10/10 |
- As observed, even with the larger subset, we are utilizing only approximately 16% of the available data.
- Important: Although we initially created an
80/10/10
split, the test split from both subsets was not used. Instead, we used a standardized held-out test set, allowing us to better understand how the size of the dataset impacts performance in a comparable manner.
To determine if fine-tuning Whisper on a Singapore English dataset would lead to improvements, we first established a baseline by evaluating the performance of the base whisper-small model on a held-out test set, which was generated from the last 100 transcripts.
- To be specific, we utilized the speaker IDs from the following set: [3109, …, 3191, 3500].
- The held-out test set contains a total of 43,788 audio samples.
This initial assessment provides a benchmark against which we can compare any improvements resulting from fine-tuning.
For our benchmark metrics, we have chosen the most commonly adopted metric for ASR tasks: Word Error Rate (WER
).
As a quick refresher, WER is the number of errors in the model’s transcripts relative to the number of words in the reference transcripts.
It is calculated as follows:
Where:
S
is the number of substitutionsD
is the number of deletionsI
is the number of insertionsN
is the total number of words in the reference
For details on the code implementation, please refer to the section on Evaluation Metrics.
Evaluated based on WER, the performance of the base model is as follows:
Model | WER |
---|---|
base-whisper-small | 57.90% |
In addition to evaluating the base model’s performance using WER, I also assessed its performance on the sample audio files provided in the previous section.
import librosafrom transformers import AutoTokenizer, pipeline
tokenizer = AutoTokenizer.from_pretrained("openai/whisper-small")
base_pipeline = pipeline( "automatic-speech-recognition", model="openai/whisper-small", tokenizer=tokenizer,)
audio_path_transcript = [ { "path": "singlish-audio-food.wav", "transcript": "Seafood Hor Fun, Soon Kueh, and Braised Shark Fin soup", }, { "path": "singlish-audio-places.wav", "transcript": "Pasir Ris Avenue, Telok Blangah Hill, and Jalan Lengkok Sembawang", }, { "path": "singlish-audio-names.wav", "transcript": "Tay Kay Chin, Wong Kan Seng and Ee Peng Liang? Are you not familiar with these names?", }, { "path": "singlish-audio-brands.wav", "transcript": "MOS burger, Kappa, and Tiffany & Co.", }, { "path": "singlish-audio-mix.wav", "transcript": "I am meeting Breanna at Ang Mo Kio Electronics Park Road first", },]
for sample in audio_path_transcript: audio, rate = librosa.load(sample["path"], sr=16000) base_result = base_pipeline(audio, generate_kwargs={"language": "english"}) print(f"Original: {sample['transcript']}") print(f"Transcribed: {base_result['text']}") print()
As you might expect, the base whisper-small model did not perform well given the unique blend of Singaporean slang and English in the audio samples:
Original: Seafood Hor Fun, Soon Kueh, and Braised Shark Fin soupTranscribed: See for what fun, soon great and breathe shark fin soup.
Original: Pasir Ris Avenue, Telok Blangah Hill, and Jalan Lengkok SembawangTranscribed: Passerys Avenue, Telok Belangah Hill and Jalan Lengkot Sembawang
Original: Tay Kay Chin, Wong Kan Seng and Ee Peng Liang? Are you not familiar with these names?Transcribed: Takei Qin, Wong Kan Sing, and Yi Bing Liang. Are you not familiar with his names?
Original: MOS burger, Kappa, and Tiffany & Co.Transcribed: Mos Burger, Kappa, and Tiffany & Co.
Original: I am meeting Breanna at Ang Mo Kio Electronics Park Road firstTranscribed: I am meeting Brianna at Mokyo Electronics Park Road first.
Developing Singlish-Whisper
There have been many fine-tuning guides for Whisper models, making the overall process quite generic. The following fine-tuning code has been primarily adapted from these sources:
- https://github.com/huggingface/community-events/blob/main/whisper-fine-tuning-event/fine-tune-whisper-non-streaming.ipynb
- https://medium.com/@bofenghuang7/what-i-learned-from-whisper-fine-tuning-event-2a68dab1862
Hence, feel free to skip the following sections if you are familiar with the process.
Load Model
The base model we are using to fine-tune for Singlish is openai/whisper-small.
To load the model, we will be using the WhisperForConditionalGeneration
class from the transformers
library:
from transformers import WhisperForConditionalGeneration
model = WhisperForConditionalGeneration.from_pretrained("openai/whisper-small")
Some adjustments were also made to the model’s configuration for the training process, including:
# disable cache during training since it's incompatible with gradient checkpointingmodel.config.use_cache = False
# set language and task for generation and re-enable cachemodel.generate = partial( model.generate, language="english", task="transcribe", forced_decoder_ids=None, use_cache=True)
- We re-enabled
use_cache=True
during generation to speed up the decoding process.
Load Dataset
The initial dataset, as downloaded from the NSC data source, will be in the following format:
.├── SCRIPT│ ├── 020010.TXT│ ├── 020011.TXT│ ├── 020020.TXT│ ├── 020021.TXT│ ├── 020030.TXT│ ├── 020031.TXT│ └── 020050.TXT└── WAVE ├── SPEAKER2001.zip ├── SPEAKER2002.zip ├── SPEAKER2003.zip └── SPEAKER2005.zip
The SCRIPT
folder contains each speaker’s transcript, denoted by the filename and session number.
Meanwhile, the WAVE
folder contains each speaker’s recordings from the sessions they participated in.
An example of a transcript is as follows:
020010001 4 four020010002 DC, Asos, and FairPrice. D C Asos and FairPrice020010003 Jingisukan, Breadsticks, and Yaki udon. ** Jingisukan Breadsticks and Yaki Udon020010004 Where can I find the best yang rou tang? where can I find the best Yang you Tang020010005 png kueh, fried garoupa, and butter pork ribs. Png Kueh Fried Garoupa and Butter Pork Ribs
The initial 9 digits denote the corresponding audio file name, and the following new line contains the actual transcription based on the speaker’s recording.
The corresponding audio file for the 5th transcript sentence is 020010005.WAV
Therefore, the process to prepare the raw dataset for training involves matching each transcript sentence with its corresponding audio file.
Please refer to the repository for the full code implementation in processing the transcript and audio.
The final dataset produced is a DatasetDict
object, which is a dictionary-like object that contains the following keys:
train
: The training dataset.validation
: The validation dataset.test
: The test dataset.
DatasetDict({ train: Dataset({ features: ['audio', 'transcript'], num_rows: 31728 }) validation: Dataset({ features: ['audio', 'transcript'], num_rows: 3966 }) test: Dataset({ features: ['audio', 'transcript'], num_rows: 3967 })})
- This is the
DatasetDict
used for the initial fine-tuning, containing 39,661 samples.
Pre-processing
As understood, Whisper models are a family of models that is based on traditional encoder-decoder Transformers architecture. This meant that we need to prepare the dataset in a way that is compatible with the model’s (encoder) input and (decoder) output.
The general pre-processing steps for the dataset involves:
- Loading the audio file and its sampling rate.
- Computing the log-Mel input features from the audio array.
- Encoding the target text to label ids 1.
def prepare_dataset(batch): """ Prepare the dataset for training.
Args: batch (Dict[str, Any]): The batch to prepare.
Returns: Dict[str, Any]: The prepared batch. """ # load audio = batch["audio"] # compute log-Mel input features from input audio array batch["input_features"] = processor.feature_extractor( audio["array"], sampling_rate=audio["sampling_rate"] ).input_features[0]
# encode target text to label ids batch["labels"] = processer.tokenizer(batch["transcript"]).input_ids
return batch
Alternatively, you can prepare both the audio and the transcript separately, using the WhisperFeatureExtractor
for the audio and the WhisperTokenizer
for the transcript.
from transformers import WhisperFeatureExtractor, WhisperTokenizer
# generate log-Mel spectogramfeature_extractor = WhisperFeatureExtractor.from_pretrained( "openai/whisper-small", language="English", task="transcribe")
# generate BPE tokenstokenizer = WhisperTokenizer.from_pretrained( "openai/whisper-small", language="English", task="transcribe" )
Training and Evaluation
Data Collator
As we will be training in batches, it is important to ensure that the input and output features are of the same length. This is achieved by padding the input features and labels to the maximum length. In addition, we will also need to ensure each input is correctly paired with its corresponding output.
As such, we create a custom data collator – DataCollatorSpeechSeq2SeqWithPadding
to handle the tasks above.
@dataclassclass DataCollatorSpeechSeq2SeqWithPadding: """ Data collator for speech seq2seq with padding. """
processor: Any decoder_start_token_id: int
def __call__( self, features: List[Dict[str, Union[List[int], torch.Tensor]]] ) -> Dict[str, torch.Tensor]: # split inputs and labels since they have to be of different lengths and need different padding methods # first treat the audio inputs by simply returning torch tensors input_features = [ {"input_features": feature["input_features"]} for feature in features ] batch = self.processor.feature_extractor.pad( input_features, return_tensors="pt" )
# get the tokenized label sequences label_features = [{"input_ids": feature["labels"]} for feature in features] # pad the labels to max length labels_batch = self.processor.tokenizer.pad(label_features, return_tensors="pt")
# replace padding with -100 to ignore loss correctly labels = labels_batch["input_ids"].masked_fill( labels_batch.attention_mask.ne(1), -100 )
# if bos token is appended in previous tokenization step, # cut bos token here as it's append later anyways if (labels[:, 0] == self.decoder_start_token_id).all().cpu().item(): labels = labels[:, 1:]
batch["labels"] = labels
return batch
- The output (
labels
) is padded to the maximum length, with padded tokens replaced by -100 to ensure that they are not factored into the loss computation. - If you are fine-tuning on a dataset with audio samples longer than 30 seconds, you may need to chunk the audio accordingly.
Evaluation Metrics
Instead of writing your own function to compute the WER metric based on the formula shown in the earlier section, you can simply use the Evaluate library:
import evaluate
metric = evaluate.load("wer")
def compute_metrics(pred) -> Dict[str, float]: """ Compute metrics for the model.
This function computes the Word Error Rate (WER) between the predicted and reference transcripts. The WER is a common metric used to evaluate the performance of automatic speech recognition (ASR) systems. It is calculated as the number of errors (insertions, deletions, and substitutions) divided by the total number of words in the reference transcript, multiplied by 100 to get a percentage.
Args: pred (transformers.EvalPrediction): The predictions and label_ids from the model.
Returns: Dict[str, float]: A dictionary containing the WER metric. """ pred_ids = pred.predictions label_ids = pred.label_ids
# replace -100 with the pad_token_id label_ids[label_ids == -100] = tokenizer.pad_token_id
# we do not want to group tokens when computing the metrics pred_str = tokenizer.batch_decode(pred_ids, skip_special_tokens=True) label_str = tokenizer.batch_decode(label_ids, skip_special_tokens=True)
wer = 100 * metric.compute(predictions=pred_str, references=label_str) # type: ignore
return {"wer": wer}
- Since we replaced the padded tokens with
-100
during the evaluation process, we will need to convert them back to the padding token (<|endoftext|>
) before computing the metrics.
Training Configuration
The initial Seq2SeqTrainingArguments
for the training process are as follows:
training_args = Seq2SeqTrainingArguments( output_dir="./whisper-small-singlish", per_device_train_batch_size=128, gradient_accumulation_steps=1, learning_rate=1e-5, warmup_steps=500, max_steps=5000, gradient_checkpointing=True, fp16=True, evaluation_strategy="steps", per_device_eval_batch_size=16, predict_with_generate=True, generation_max_length=225, save_steps=500, eval_steps=500, logging_steps=500, load_best_model_at_end=True, metric_for_best_model="wer", greater_is_better=False, push_to_hub=False, max_grad_norm=1.0,)
Further adjustments were made to the training arguments to optimize the training process as we initiated the training run.
Training Run
The following arguments for Seq2SeqTrainer
are used:
trainer = Seq2SeqTrainer( args=training_args, model=model, train_dataset=vectorized_datasets["train"], eval_dataset=vectorized_datasets["validation"], data_collator=data_collator, compute_metrics=compute_metrics, tokenizer=processor.feature_extractor,)
trainer.train()
For training, I am using Jarvislabs with a single A6000 GPU.
Generally, I will leave the training process as it is, understanding that it can take a considerable amount of time. However, for the first 1,000 steps, I closely monitor the training metrics to ensure the model is learning effectively and to make optimal use of the hardware resources.
During the initial training run for subset 1, we began with a batch size of 32
.
Upon observing that overall memory utilization remained relatively low, I increased the batch size to 64
for the remainder of the training.
Based on the overall training time and my understanding of the process from subset 1, I further increased the training batch size to 128
and set the evaluation batch size to 32
for the rest of the training for subset 2.
In addition, I prematurely stopped the training process (for subset 2) when I observed that the evaluation loss had plateaued and signs of overfitting were present.
A rough estimate of the training time for subset 1 and subset 2 is as follows:
Subset | Training Time | Steps |
---|---|---|
Subset 1 | 6-7 hours | 5,000 |
Subset 2 | 29-30 hours | 4,000 |
Taking into account the data transfer and model training time, the overall training cost was approximately $50.
To further optimize the cost, consider the following strategies:
- Only Transfer Necessary Data: Instead of transferring the entire dataset, which in my case was around 100GB, transfer only the data you need. This can significantly reduce the data transfer time and associated costs.
- Use
EarlyStoppingCallback
and Auto-Pause Features: Implementing the EarlyStoppingCallback can help stop the training early if the model’s performance stops improving. Additionally, using an auto-pause feature for your instance can prevent incurring further costs when the instance is idle, saving both time and money.
Results
TLDR: If you are not interested in the specifics, the final results compared to the base whisper-small model are as follows:
Model | WER |
---|---|
base-whisper-small | 57.90% |
whisper-small-singlish-39k | 13.59% |
whisper-small-singlish-122k | 9.69% |
Subset 1
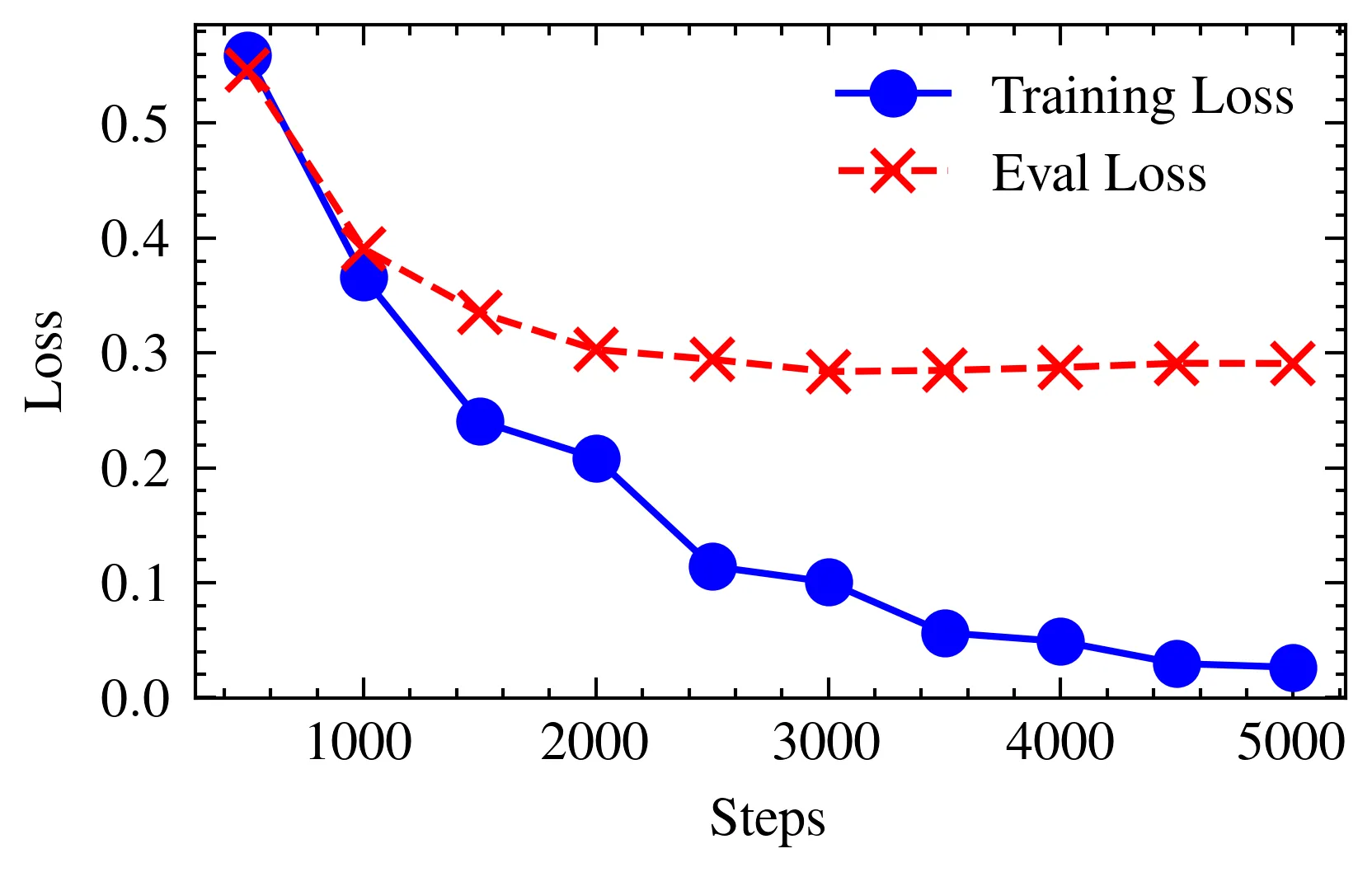
In the initial steps, the average training loss is high but drops significantly till 2,000 steps, indicating rapid initial learning. From the 2,000th step, the decline slows, and the training loss continues to decrease, approaching near zero by the 5,000th step.
The evaluation loss follows a similar trend, with the most substantial reduction occurring within the first 1,000 steps. The lowest evaluation loss is observed at the 3,000th step, with a loss of 0.28369.
However, beyond the 3,000th step, the evaluation loss begins to rise slightly. In addition, we started to see a divergence between the training and evaluation loss from the 2,000th step onwards, signalling potential overfitting.
- We observe the training loss continually decreasing, while the evaluation loss plateaus.
- This may indicate that our model is overly specialized in fitting the training data, as explained here.
- However, we also observed that even though the evaluation loss has plateaued, the WER is still decreasing, albeit at a slower rate.
Based on my research, the general guideline is to fine-tune for no more than 2-4 epochs. Therefore, ensure to regularly checkpoint within this range to capture the best model.
Step | Eval Loss | Eval WER (%) |
---|---|---|
500 | 0.546262 | 23.49% |
1000 | 0.390565 | 17.86% |
1500 | 0.334905 | 15.61% |
2000 | 0.302827 | 13.90% |
2500 | 0.294299 | 13.41% |
3000 | 0.283697 | 13.09% |
3500 | 0.284752 | 12.88% |
4000 | 0.287253 | 12.85% |
4500 | 0.290913 | 12.73% |
5000 | 0.290750 | 12.64% |
The WER starts at 23.49% initially, indicating a high number of prediction errors with the Singlish dataset. As training progresses, WER decreases significantly, showing noticeable improvement by the midpoint of training.
After evaluating the loss curves, we selected the checkpoint at the 2,000th step as the final model. This checkpoint demonstrated the best balance where the training loss is still relatively low and the validation loss has not yet started to rise.
The selection of the best model takes into account its performance on both training and validation data. A model that performs similarly well on both is more likely to generalize effectively to new, unseen data.
To truly understand the model’s performance, we evaluated it on the held-out test set. The performance is as follows:
Model | WER |
---|---|
whisper-small-singlish-39k | 13.59% |
Comparing to the initial performance of base whisper-small, the fine-tuned model demonstrated a significant improvement in WER from 57.90% to 13.59% (~4x better)
In addition, when evaluated on the sample audio files, the model demonstrated significantly better transcription performance compared to the base model.
Original: Seafood Hor Fun, Soon Kueh, and Braised Shark Fin soupTranscribed: Seafood Hor Fun Soon Kueh and Braised Shark Fin Soup
Original: Pasir Ris Avenue, Telok Blangah Hill, and Jalan Lengkok SembawangTranscribed: Pasir Race Avenue Telok Blangah Hill and Jalan Lengkok Sembawang
Original: Tay Kay Chin, Wong Kan Seng and Ee Peng Liang? Are you not familiar with these names?Transcribed: Tay Kay Chin Wong Kan Seng and Yee Peng Liang are you not familiar with these names
Original: MOS burger, Kappa, and Tiffany & Co.Transcribed: Mos Burger Kappa and Tiffanie and Co
Original: I am meeting Breanna at Ang Mo Kio Electronics Park Road firstTranscribed: I am meeting Brianna at Mokio Electronics Park Road first
- The fine-tuned whisper-small model demonstrates improved accuracy in capturing both Chinese names and street names compared to the base model.
- However, some other more prominent issues surfaced, particularly with brand names. For instance, the brand name
Tiffany & Co.
is incorrectly transcribed asTiffanie and Co
.
Subset 2
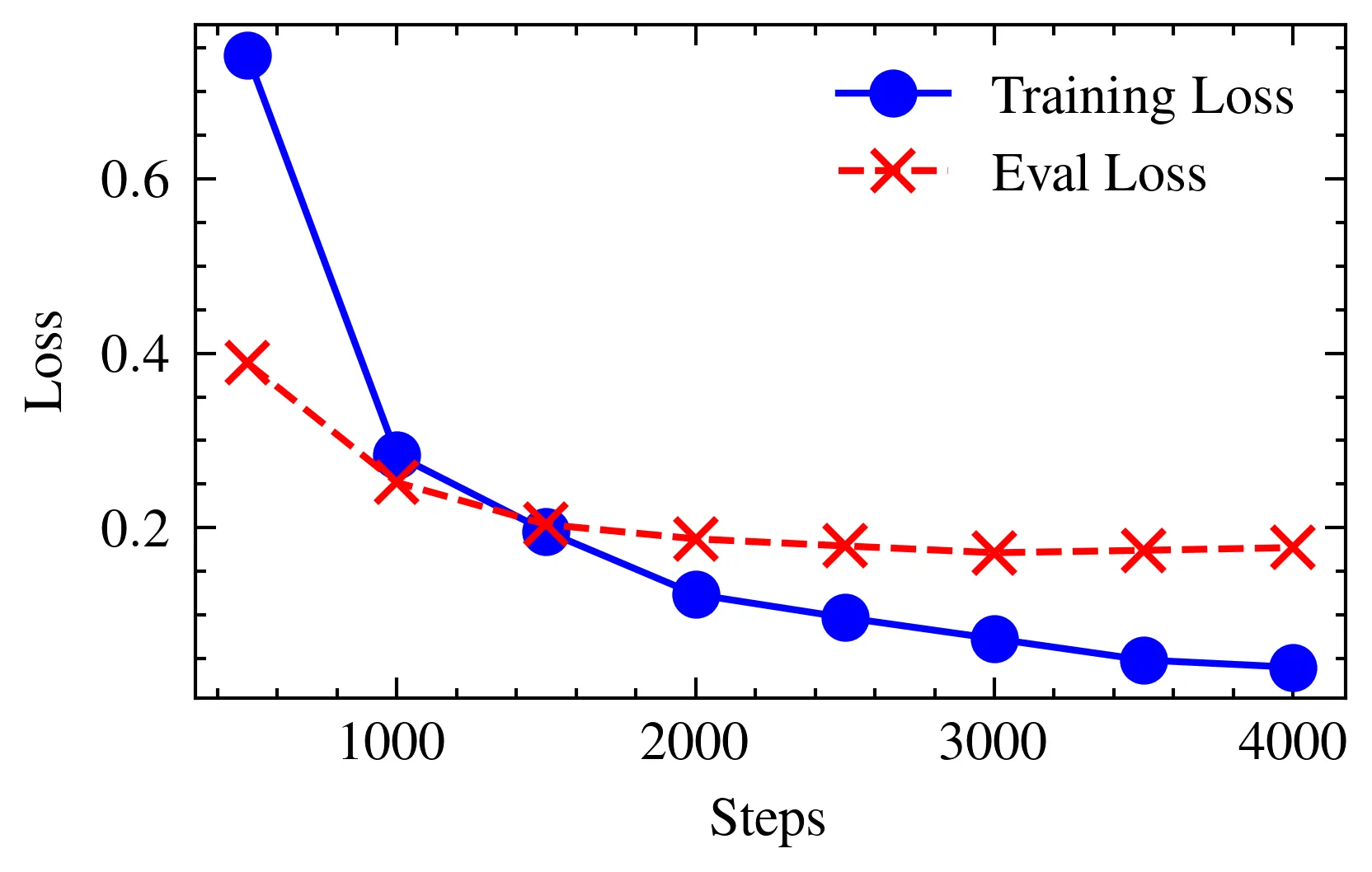
In the initial steps, the average training loss is high but drops significantly until around the 1,000th step, indicating rapid initial learning. From the 1,000th to the 2,000th step, the decline in training loss continues but at a slower rate. Beyond 2,000 steps, the reduction in training loss becomes more gradual, approaching near zero by the 4,000th step.
The evaluation loss exhibits a similar trend initially, with a substantial reduction within the first 1,000 steps. The lowest evaluation loss is observed around the 3,000th step. Generally, we can see that the evaluation loss starts to plateau from around 1,500 steps onwards. Although it remains relatively stable, there is a slight increase in evaluation loss beyond the 3,000th step.
From around the 2,000th step onwards, a divergence between training and evaluation loss becomes noticeable, which suggests potential overfitting. We did not observe a significant divergence between the evaluation loss and the training loss, unlike what was seen during the training of subset 1.
In general, we observe a similar training pattern for both subsets. However, with more data, the model demonstrates an improved ability to generalize to unseen data.
Step | Eval Loss | Eval WER (%) |
---|---|---|
500 | 0.388949 | 17.97% |
1000 | 0.251898 | 11.88% |
1500 | 0.203766 | 9.95% |
2000 | 0.187231 | 9.42% |
2500 | 0.179108 | 8.54% |
3000 | 0.171377 | 8.21% |
3500 | 0.174112 | 8.15% |
4000 | 0.177270 | 8.14% |
The WER pattern is similar to what we observed in subset 1: the WER started high and decreased significantly. However, after mid-training, the improvements in WER became less significant.
As expected, the WER with the larger dataset is lower compared to the first training run.
Considering the potential overfitting based on the loss curves, we selected the checkpoint at the 3,000th step as the final model. This checkpoint demonstrated the best balance, with the training loss still relatively low and the evaluation loss at its lowest value of 0.171377.
When evaluated on the held-out test set, we observed better performance compared to subset 1. This outcome answers our objective of running the fine-tuning process on two differently-sized datasets: yes, more data results in better fine-tuning performance.
Model | WER |
---|---|
whisper-small-singlish-122k | 9.69% |
Comparing to the initial performance of base whisper-small, the fine-tuned model demonstrated a significant improvement in WER from 57.90% to 9.69% (~6x better). Relative to the performance when fine-tuning on ~39k audio samples, using around ~122k audio samples results in a further improvement of +3.9%.
In the original paper, the scaling of the dataset was also explored. It was observed that increases in dataset size generally improve performance across all tasks.
We also repeated the same evaluation on the audio samples, where the model demonstrated further improvements compared to subset 1:
Original: Seafood Hor Fun, Soon Kueh, and Braised Shark Fin soupTranscribed: Seafood Hor Fun Soon Kuih and Braised Shark Fin Soup
Original: Pasir Ris Avenue, Telok Blangah Hill, and Jalan Lengkok SembawangTranscribed: Pasir Ris Avenue Telok Blangah Hill and Jalan Lengkok Sembawang
Original: Tay Kay Chin, Wong Kan Seng and Ee Peng Liang? Are you not familiar with these names?Transcribed: Tay Kay Chin Wong Kan Seng and Ee Peng Liang are you not familiar with these names
Original: MOS burger, Kappa, and Tiffany & Co.Transcribed: Mos Burger Kappa and Tiffany Anchor
Original: I am meeting Breanna at Ang Mo Kio Electronics Park Road firstTranscribed: I am meeting Breanna at Mokio Electronics Park Road first
- Compared to subset 1, the model improved in capturing both Chinese names and street names.
- The term
kueh
as per the original transcript is incorrectly transcribed askuih
, however both terms are interchangeably used in the Singapore context. - Likewise in subset 1, the brand name
Tiffany & Co.
is still incorrectly transcribed, but this time asTiffany Anchor
.
Simple Qualitative Assessment
In addition to the quantitative assessment of the fine-tuned model, I decided to understand its performance in practical scenarios. Specifically, I look into testing the model’s ability to transcribe audio recordings spoken in Singlish.
Transcript: Eh brother, you know yesterday night [ah], I was really very hungry [eh]. So I decided to go downstairs and buy Bak Chor Mee to eat. But then, I don’t know why [ah], the Bak Chor Mee aunty look at me like I some siao gina. Maybe, I don’t think [ah] she like me, how [ah]?
- Bak Chor Mee: Minced Meat Noodles, a traditional dish in Singapore.
- Siao Gina: The term “Siao” means crazy and “Gina” (gín-á) means child, both of which originated from the Hokkien dialect. Usually used in the context of describing someone acting or behaving out of the norm.
The audio clip I recorded is something I might say to my friends, and it would be considered Singlish.
Let’s take a look at how the base model and openapi/whisper-large-v3 and the fine-tuned model perform:
whisper-small
:
100:00:00,000 --> 00:00:07,600Hey brother, you know yesterday night I was very very hungry so I decided to go downstairs and buy a bottle me do it
200:00:07,680 --> 00:00:12,440But then I don't know why the bottom me auntie look at me like some silky nah
300:00:12,720 --> 00:00:15,480Maybe I don't think she like me. How ah?
whisper-large-v3
:
100:00:00,000 --> 00:00:05,000Hey brother, you know yesterday night ah, I was very very hungry eh.
200:00:05,000 --> 00:00:08,000So I decided to go downstairs and buy bak chor mee to eat.
300:00:08,000 --> 00:00:13,000But then, I don't know why ah, the bak chor mee auntie look at me like I'm some xiao gina ah.
400:00:13,000 --> 00:00:16,184Maybe I don't think eh, she like me. How ah?
fine-tuned-39k-whisper-small
:
100:00:00,000 --> 00:00:05,380Hey brother you know yesterday night I was very very hungry so I
200:00:05,380 --> 00:00:09,380decided to go downstairs and buy Batok Medu Yat Ba Den I don't know
300:00:09,380 --> 00:00:13,460why the Batok Med Aunty look at me like I'm some Seow Gina maybe I
400:00:13,460 --> 00:00:17,420don't think she likes me how are
fine-tuned-122k-whisper-small
:
100:00:00,000 --> 00:00:05,340hey brother you know yesterday night I was very very hungry so I
200:00:05,340 --> 00:00:09,240decided to go downstairs and buy Bak Chor Mee to eat but then I don't
300:00:09,240 --> 00:00:12,440know why the Bak Chor Mee Auntie look at me like I some Seow Gina
400:00:12,440 --> 00:00:15,480and maybe I don't think and she like me how are
While this demonstration may not be significant, it is interesting to note that the fine-tuned model(s) performed better than the base whisper-small model in capturing local words and terms!
Due to my resource limitations, this exploration largely skipped the bigger and more capable Whisper models. Hence, when we examined the performance of whisper-large-v3 in this small demonstration, it was surprising to see how well it handled Singlish. In fact, I would personally say that the transcript was better than the ones we fine-tuned and the closest to my own recording.
Nevertheless, we still need to consider that the number of parameters in whisper-large-v3 is approximately 6x larger than in whisper-small, which results in higher costs and longer inference times.
From the transcript, it also appears that the fine-tuned model loses some of its ability to apply punctuation correctly.
Limitations
While the short demonstration in the previous section was interesting, there are still some limitations to this fine-tuning exploration that should be addressed:
- Length of Audio Data: The model was trained on short audio samples, typically derived from prompt-read speech data. This limited the model’s exposure to longer, naturally occurring speech patterns.
- Quality of Audio Data: The audio data was collected in a controlled environment, resulting in higher quality recordings compared to more natural scenarios, such as everyday conversations in public settings or phone calls. Consequently, the model may struggle with audio that contains more background noise or lower clarity.
Although the fine-tuning process produced WER that is lower than the base model, the model may still struggled in situation where audio quality may not be as good (e.g., public settings with background noises). Moreover, the model may struggle with natural speech patterns found in conversational dialogues, as these can present different challenges compared to the more controlled, prompt-read speech data on which the model was primarily trained.
Considering that we evaluated the process using a “clean” prompted read speech dataset, the fine-tuned model may not perform well in other contexts.
We must also consider that Singlish comes in many forms and variations, and the model may struggle with variations in pronunciation, orthography, and vocabulary.
For example, in the following video, you’ll notice that Singlish can be challenging to understand when spoken in a “broken” manner with minimal sentence structure. It’s only comprehensible if you’re familiar with the specific words being used.
If we were to fine-tune a more robust model, the audio samples used should include variations in speech format (e.g., conversations, call center), which are already present in NSC. Additionally, one might consider further data augmentation by adding various types of noise and transformations to help the model generalize better across different settings and speakers. For more information, you can refer here.
Hugging Face Hub and Spaces
Beyond my own exploration, I have also released fine-tuned-122k-whisper-small Hugging Face Hub for you to try out!

whisper-small-singlish-122k
on Hugging Face Hub
In addition, I have created a Hugging Face Space to allow easier interactions with the model.
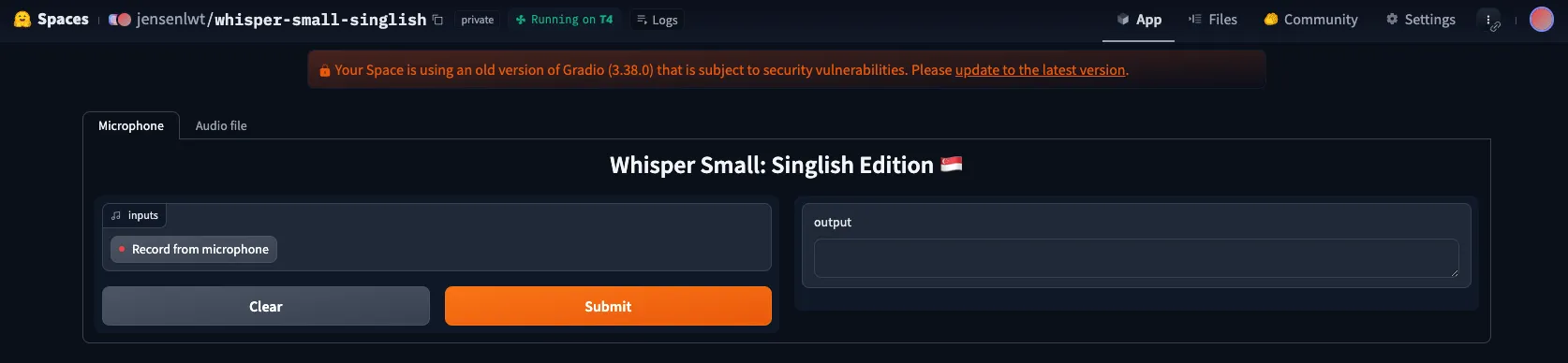
whisper-small-singlish-122k demo on Hugging Face Hub
Conclusion
It was fun to explore fine-tuning models after a while of working with LLM operations, especially on a topic that strongly resonates with me as a Singaporean.
Throughout the whole process, we witnessed how we can improve the performance of the base whisper-small model on an arguably low-resource “language” such as Singlish. Although the numbers shown here indicate an improvement, the models we fine-tuned exhibited behaviors that may not be ideal.
For example, they started to mis-transcribe words they originally handled well, such as transcribing Tiffany & Co.
to Tiffanie Anchor
.
In the section on qualitative assessment, we observed that, even without fine-tuning, the largest and best Whisper model can already perform a superb job in transcribing Singlish.
While the large Whisper model offers robust performance, there are compelling reasons to fine-tune smaller, faster, and more cost-effective Whisper models for specialized tasks.
For example, in a call center scenarios within the Singapore context, fine-tuning smaller Whisper models on data specifically collected in Singapore can significantly improve their ability to handle different Singapore pronunciation and the unique characteristics of Singlish terms in a cost-efficient manner.
However, to save time and effort, it is often advisable to use the original model unless your specific scenario necessitates significantly improved performance due to unique circumstances.
In the near future, I would like to explore more efficient methods of fine-tuning models, such as using PEFT and the distilled Whisper model. PEFT fine-tuning techniques enable more efficient fine-tuning in a shorter time while still achieving comparable results. Meanwhile, the distilled models have shown performance similar to the base models but are smaller in size.
Till next time! 🚀
Resources
- https://huggingface.co/blog/fine-tune-whisper
- https://medium.com/@bofenghuang7/what-i-learned-from-whisper-fine-tuning-event-2a68dab1862
- https://huggingface.co/spaces/openai/whisper/discussions/6#63c5731dfb9a6b829d898bc8
- https://wandb.ai/mostafaibrahim17/ml-articles/reports/A-Deep-Dive-Into-Learning-Curves-in-Machine-Learning—Vmlldzo0NjA1ODY0
- https://datascience.stackexchange.com/a/42600
- https://www.assemblyai.com/blog/how-to-run-openais-whisper-speech-recognition-model/#openai-whisper-analysis
Footnotes
-
During the fine-tuning process, I neglected the important step of thoroughly exploring the transcript of the raw dataset. As a result, some minor issues arose, such as:
- The transcript used special terms to denote certain words when the voice was inaudible to the transcriber or filler words.
- E.g.,
<FIL/>
for fillers,**
for unintelligible words - In addition, acronyms were spelled out in the transcript with spaces separating each letter.
- E.g.,
GST
(Goods and Services Tax) is spelled asG S T
- The transcriber also made careless mistakes, such as:
- E.g.,
Where can I find the best yang rou tang?
is transcribed aswhere can I find the best Yang you Tang